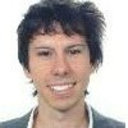
Mirco RavanelliConcordia University Montreal · Department of Computer Science and Software Engineering
Mirco Ravanelli
PhD
Deep learning, conversational AI, and EEG signal processing. Creator of SpeechBrain (https://speechbrain.github.io/)
About
112
Publications
46,491
Reads
How we measure 'reads'
A 'read' is counted each time someone views a publication summary (such as the title, abstract, and list of authors), clicks on a figure, or views or downloads the full-text. Learn more
4,354
Citations
Introduction
I'm an Assistant Professor at Concordia University (Gina Cody School of Engineering and Computer Science) working on deep learning for sequence processing, with a focus on Conversational AI. I'm also adjunct professor at the Université de Montréal (DIRO - Département d'informatique et de recherche opérationnelle) and Associate Member at Mila - Quebec AI Institute.
I'm the author of more than 50 papers on deep learning, conversational AI, and EEG signal processing.
Additional affiliations
Education
September 2005 - February 2011
Publications
Publications (112)
Discrete audio tokens have recently gained considerable attention for their potential to connect audio and language processing, enabling the creation of modern multimodal large language models. Ideal audio tokens must effectively preserve phonetic and semantic content along with paralinguistic information, speaker identity, and other details. While...
Discrete audio tokens have recently gained attention for their potential to bridge the gap between audio and language processing. Ideal audio tokens must preserve content, paralinguistic elements, speaker identity, and many other audio details. Current audio tokenization methods fall into two categories: Semantic tokens, acquired through quantizati...
In this paper, we propose Phoneme Discretized Saliency Maps (PDSM), a discretization algorithm for saliency maps that takes advantage of phoneme boundaries for explainable detection of AI-generated voice. We experimentally show with two different Text-to-Speech systems (i.e., Tacotron2 and Fastspeech2) that the proposed algorithm produces saliency...
Interpreting the decisions of deep learning models, including audio classifiers, is crucial for ensuring the transparency and trustworthiness of this technology. In this paper, we introduce LMAC-ZS (Listenable Maps for Audio Classifiers in the Zero-Shot context), which, to the best of our knowledge, is the first decoder-based post-hoc interpretatio...
p>This paper introduces Continual Learning for Multilingual ASR (CL-MASR), a benchmark for continual learning applied to multilingual ASR. CL-MASR offers a curated selection of medium/low-resource languages, a modular and flexible platform for executing and evaluating various CL methods on top of existing large-scale pretrained multilingual ASR mod...
p>This paper introduces Continual Learning for Multilingual ASR (CL-MASR), a benchmark for continual learning applied to multilingual ASR. CL-MASR offers a curated selection of medium/low-resource languages, a modular and flexible platform for executing and evaluating various CL methods on top of existing large-scale pretrained multilingual ASR mod...
Self-supervised learning (SSL) leverages large datasets of unlabeled speech to reach impressive performance with reduced amounts of annotated data. The high number of proposed approaches fostered the emergence of comprehensive benchmarks that evaluate their performance on a set of downstream tasks exploring various aspects of the speech signal. How...
Speech Emotion Recognition (SER) typically relies on utterance-level solutions. However, emotions conveyed through speech should be considered as discrete speech events with definite temporal boundaries, rather than attributes of the entire utterance. To reflect the fine-grained nature of speech emotions, we propose a new task: Speech Emotion Diari...
Self-supervised learning (SSL) has recently allowed leveraging large datasets of unlabeled speech signals to reach impressive performance on speech tasks using only small amounts of annotated data. The high number of proposed approaches fostered the need and rise of extended benchmarks that evaluate their performance on a set of downstream tasks ex...
In this paper, we introduce a new approach, called "Posthoc Interpretation via Quantization (PIQ)", for interpreting decisions made by trained classifiers. Our method utilizes vector quantization to transform the representations of a classifier into a discrete, class-specific latent space. The class-specific codebooks act as a bottleneck that force...
Self-supervised learning (SSL) has allowed substantial progress in Automatic Speech Recognition (ASR) performance in low-resource settings. In this context, it has been demonstrated that larger self-supervised feature extractors are crucial for achieving lower downstream ASR error rates. Thus, better performance might be sanctioned with longer infe...
Transformers have enabled impressive improvements in deep learning. They often outperform recurrent and convolutional models in many tasks while taking advantage of parallel processing. Recently, we proposed the SepFormer, which obtains state-of-the-art performance in speech separation with the WSJ0-2/3 Mix datasets. This paper studies in-depth Tra...
End-to-end speech synthesis models directly convert the input characters into an audio representation (e.g., spectrograms). Despite their impressive performance, such models have difficulty disambiguating the pronunciations of identically spelled words. To mitigate this issue, a separate Grapheme-to-Phoneme (G2P) model can be employed to convert th...
Transformers have recently achieved state-of-the-art performance in speech separation. These models, however, are computationally-demanding and require a lot of learnable parameters. This paper explores Transformer-based speech separation with a reduced computational cost. Our main contribution is the development of the Resource-Efficient Separatio...
In this paper, we present a self-supervised learning framework for continually learning representations for new sound classes. The proposed system relies on a continually trained neural encoder that is trained with similarity-based learning objectives without using labels. We show that representations learned with the proposed method generalize bet...
Transformers have enabled major improvements in deep learning. They often outperform recurrent and convolutional models in many tasks while taking advantage of parallel processing. Recently, we have proposed SepFormer, which uses self-attention and obtains state-of-the art results on WSJ0-2/3 Mix datasets for speech separation. In this paper, we ex...
In this paper, we work on a sound recognition system that continually incorporates new sound classes. Our main goal is to develop a framework where the model can be updated without relying on labeled data. For this purpose, we propose adopting representation learning, where an encoder is trained using unlabeled data. This learning framework enables...
Although deep learning (DL) has achieved notable progress in speech enhancement (SE), further research is still required for a DL-based SE system to adapt effectively and efficiently to particular speakers. In this study, we propose a novel meta-learning-based speaker-adaptive SE approach (called OSSEM) that aims to achieve SE model adaptation in a...
In recent years, deep learning based source separation has achieved impressive results. Most studies, however, still evaluate separation models on synthetic datasets, while the performance of state-of-the-art techniques on in-the-wild speech data remains an open question. This paper contributes to fill this gap in two ways. First, we release the RE...
Most of the deep learning-based speech enhancement models are learned in a supervised manner, which implies that pairs of noisy and clean speech are required during training. Consequently, several noisy speeches recorded in daily life cannot be used to train the model. Although certain unsupervised learning frameworks have also been proposed to sol...
Machine learning methods, such as deep learning, show promising results in the medical domain. However, the lack of interpretability of these algorithms may hinder their applicability to medical decision support systems. This paper studies an interpretable deep learning technique, called SincNet. SincNet is a convolutional neural network that effic...
Machine learning methods, such as deep learning, show promising results in the medical domain. However, the lack of interpretability of these algorithms may hinder their applicability to medical decision support systems. This paper studies an interpretable deep learning technique, called SincNet. SincNet is a convolutional neural network that effic...
SpeechBrain is an open-source and all-in-one speech toolkit. It is designed to facilitate the research and development of neural speech processing technologies by being simple, flexible, user-friendly, and well-documented. This paper describes the core architecture designed to support several tasks of common interest, allowing users to naturally co...
This paper introduces Timers and Such, a new open source dataset of spoken English commands for common voice control use cases involving numbers. We describe the gap in existing spoken language understanding datasets that Timers and Such fills, the design and creation of the dataset, and experiments with a number of ASR-based and end-to-end baselin...
Learning robust speaker embeddings is a crucial step in speaker diarization. Deep neural networks can accurately capture speaker discriminative characteristics and popular deep embeddings such as x-vectors are nowadays a fundamental component of modern diarization systems. Recently, some improvements over the standard TDNN architecture used for x-v...
An important development in deep learning from the earliest MLPs has been a move towards architectures with structural inductive biases which enable the model to keep distinct sources of information and routes of processing well-separated. This structure is linked to the notion of independent mechanisms from the causality literature, in which a mec...
Despite the significant progress in automatic speech recognition (ASR), distant ASR remains challenging due to noise and reverberation. A common approach to mitigate this issue consists of equipping the recording devices with multiple microphones that capture the acoustic scene from different perspectives. These multi-channel audio recordings conta...
Recurrent Neural Networks (RNNs) have long been the dominant architecture in sequence-to-sequence learning. RNNs, however, are inherently sequential models that do not allow parallelization of their computations. Transformers are emerging as a natural alternative to standard RNNs, replacing recurrent computations with a multi-head attention mechani...
This paper introduces BIRD, the Big Impulse Response Dataset. This open dataset consists of 100,000 multichannel room impulse responses (RIRs) generated from simulations using the Image Method, making it the largest multichannel open dataset currently available. These RIRs can be used toperform efficient online data augmentation for scenarios that...
Despite the significant progress in automatic speech recognition (ASR), distant ASR remains challenging due to noise and reverberation. A common approach to mitigate this issue consists of equipping the recording devices with multiple microphones that capture the acoustic scene from different perspectives. These multi-channel audio recordings conta...
Despite the growing interest in unsupervised learning, extracting meaningful knowledge from unlabelled audio remains an open challenge. To take a step in this direction, we recently proposed a problem-agnostic speech encoder (PASE), that combines a con-volutional encoder followed by multiple neural networks, called workers, tasked to solve self-sup...
Despite the growing interest in unsupervised learning, extracting meaningful knowledge from unlabelled audio remains an open challenge. To take a step in this direction, we recently proposed a problem-agnostic speech encoder (PASE), that combines a convolutional encoder followed by multiple neural networks, called workers, tasked to solve self-supe...
This report summarizes activities and achievements obtained during and after JSALT 2019 workshop on Using Cooperative Ad-hoc Microphone Arrays for ASR. Besides its contents, relevant contributions are given by the attached slides, used during the closing ceremony, and by recent paper submissions to ICASSP 2020. The report is organized in six sectio...
End-to-end models are an attractive new approach to spoken language understanding (SLU) in which the meaning of an utterance is inferred directly from the raw audio without employing the standard pipeline composed of a separately trained speech recognizer and natural language understanding module. The downside of end-to-end SLU is that in-domain sp...
Recurrent neural networks (RNNs) are powerful architectures to model sequential data, due to their capability to learn short and long-term dependencies between the basic elements of a sequence. Nonetheless, popular tasks such as speech or images recognition, involve multi-dimensional input features that are characterized by strong internal dependen...
Learning good representations without supervision is still an open issue in machine learning, and is particularly challenging for speech signals, which are often characterized by long sequences with a complex hierarchical structure. Some recent works, however, have shown that it is possible to derive useful speech representations by employing a sel...
Whereas conventional spoken language understanding (SLU) systems map speech to text, and then text to intent, end-to-end SLU systems map speech directly to intent through a single trainable model. Achieving high accuracy with these end-to-end models without a large amount of training data is difficult. We propose a method to reduce the data require...
Whereas conventional spoken language understanding (SLU) systems map speech to text, and then text to intent, end-to-end SLU systems map speech directly to intent through a single trainable model. Achieving high accuracy with these end-to-end models without a large amount of training data is difficult. We propose a method to reduce the data require...
Learning good representations without supervision is still an open issue in machine learning, and is particularly challenging for speech signals, which are often characterized by long sequences with a complex hierarchical structure. Some recent works, however, have shown that it is possible to derive useful speech representations by employing a sel...
Deep neural networks can learn complex and abstract representations, that are progressively obtained by combining simpler ones. A recent trend in speech and speaker recognition consists in discovering these representations starting from raw audio samples directly. Differently from standard hand-crafted features such as MFCCs or FBANK, the raw wavef...
Neural network architectures are at the core of powerful automatic speech recognition systems (ASR). However, while recent researches focus on novel model architectures, the acoustic input features remain almost unchanged. Traditional ASR systems rely on multidimensional acoustic features such as the Mel filter bank energies alongside with the firs...
Learning good representations is of crucial importance in deep learning. Mutual Information (MI) or similar measures of statistical dependence are promising tools for learning these representations in an unsupervised way. Even though the mutual information between two random variables is hard to measure directly in high dimensional spaces, some rec...
Learning good representations is of crucial importance in deep learning. Mutual Information (MI) or similar measures of statistical dependence are promising tools for learning these representations in an unsupervised way. Even though the mutual information between two random variables is hard to measure directly in high dimensional spaces, some rec...
Deep learning is currently playing a crucial role toward higher levels of artificial intelligence. This paradigm allows neural networks to learn complex and abstract representations, that are progressively obtained by combining simpler ones. Nevertheless , the internal "black-box" representations automatically discovered by current neural architect...
Deep learning is currently playing a crucial role toward higher levels of artificial intelligence. This paradigm allows neural networks to learn complex and abstract representations, that are progressively obtained by combining simpler ones. Nevertheless, the internal "black-box" representations automatically discovered by current neural architectu...
Neural network architectures are at the core of powerful automatic speech recognition systems (ASR). However, while recent researches focus on novel model architectures, the acoustic input features remain almost unchanged. Traditional ASR systems rely on multidimensional acoustic features such as the Mel filter bank energies alongside with the firs...
The availability of open-source software is playing a remarkable role in the popularization of speech recognition and deep learning. Kaldi, for instance, is nowadays an established framework used to develop state-of-the-art speech recognizers. PyTorch is used to build neural networks with the Python language and has recently spawn tremendous intere...
The availability of open-source software is playing a remarkable role in the popularization of speech recognition and deep learning. Kaldi, for instance, is nowadays an established framework used to develop state-of-the-art speech recognizers. PyTorch is used to build neural networks with the Python language and has recently spawn tremendous intere...
Online speech recognition is crucial for developing natural human-machine interfaces. This modality, however, is significantly more challenging than off-line ASR, since real-time/low-latency constraints inevitably hinder the use of future information, that is known to be very helpful to perform robust predictions. A popular solution to mitigate thi...
Deep learning is progressively gaining popularity as a viable alternative to i-vectors for speaker recognition. Promising results have been recently obtained with Convolutional Neural Networks (CNNs) when fed by raw speech samples directly. Rather than employing standard hand-crafted features, the latter CNNs learn low-level speech representations...
Deep learning is progressively gaining popularity as a viable alternative to i-vectors for speaker recognition. Promising results have been recently obtained with Convolutional Neural Networks (CNNs) when fed by raw speech samples directly. Rather than employing standard hand-crafted features, the latter CNNs learn low-level speech representations...
Recurrent neural networks (RNNs) are powerful architectures to model sequential data, due to their capability to learn short and long-term dependencies between the basic elements of a sequence. Nonetheless, popular tasks such as speech or images recognition, involve multi-dimensional input features that are characterized by strong internal dependen...
Distant speech recognition is being revolutionized by deep learning, that has contributed to significantly outperform previous HMM-GMM systems. A key aspect behind the rapid rise and success of DNNs is their ability to better manage large time contexts. With this regard, asymmetric context windows that embed more past than future frames have been r...
Online speech recognition is crucial for developing natural human-machine interfaces. This modality, however, is significantly more challenging than off-line ASR, since real-time/low-latency constraints inevitably hinder the use of future information, that is known to be very helpful to perform robust predictions. A popular solution to mitigate thi...
A field that has directly benefited from the recent advances in deep learning is automatic speech recognition (ASR). Despite the great achievements of the past decades, however, a natural and robust human–machine speech interaction still appears to be out of reach, especially in challenging environments characterized by significant noise and reverb...
Deep learning is an emerging technology that is considered one of the most promising directions for reaching higher levels of artificial intelligence. Among the other achievements, building computers that understand speech represents a crucial leap towards intelligent machines. Despite the great efforts of the past decades, however, a natural and r...
Deep learning is an emerging technology that is considered one of the most promising directions for reaching higher levels of artificial intelligence.
Among the other achievements, building computers that understand speech represents a crucial leap towards intelligent machines.
Despite the great efforts of the past decades, however, a natural and r...
The availability of realistic simulated corpora is of key importance for the future progress of distant speech recognition technology. The reliability, flexibility and low computational cost of a data simulation process may ultimately allow researchers to train, tune and test different techniques in a variety of acoustic scenarios, avoiding the lab...
Audio-based multimedia retrieval tasks may identify semantic information in audio streams, i.e., audio concepts (such as music, laughter, or a revving engine). Conventional Gaussian-Mixture-Models have had some success in classifying a reduced set of audio concepts. However, multi-class classification can benefit from context window analysis and th...
Despite the significant progress made in the last years, state-of-the-art speech recognition technologies provide a satisfactory performance only in the close-talking condition. Robustness of distant speech recognition in adverse acoustic conditions, on the other hand, remains a crucial open issue for future applications of human-machine interactio...
This paper introduces the contents and the possible usage of the DIRHA-ENGLISH multi-microphone corpus, recently realized under the EC DIRHA project. The reference scenario is a domestic environment equipped with a large number of microphones and microphone arrays distributed in space. The corpus is composed of both real and simulated material, and...
Speech recognition is largely taking advantage of deep learning, showing that substantial benefits can be obtained by modern Recurrent Neural Networks (RNNs). The most popular RNNs are Long Short-Term Memory (LSTMs), which typically reach state-of-the-art performance in many tasks thanks to their ability to learn long-term dependencies and robustne...
Improving distant speech recognition is a crucial step towards flexible human-machine interfaces. Current technology, however, still exhibits a lack of robustness, especially when adverse acoustic conditions are met. Despite the significant progress made in the last years on both speech enhancement and speech recognition, one potential limitation o...
Despite the remarkable progress recently made in distant speech recognition, state-of-the-art technology still suffers from a lack of robustness, especially when adverse acoustic conditions characterized by non-stationary noises and reverberation are met. A prominent limitation of current systems lies in the lack of matching and communication betwe...
Despite the remarkable progress recently made in distant speech recognition, state-of-the-art technology still suffers from a lack of robustness, especially when adverse acoustic conditions characterized by non-stationary noises and reverberation are met. A prominent limitation of current systems lies in the lack of matching and communication betwe...
Improving distant speech recognition is a crucial step towards flexible human-machine interfaces. Current technology, however, still exhibits a lack of robustness, especially when adverse acoustic conditions are met. Despite the significant progress made in the last years on both speech enhancement and speech recognition, one potential limitation o...
Multimedia Event Detection (MED) aims to identify events—also called scenes—in videos, such as a flash mob or a wedding ceremony. Audio content information complements cues such as visual content and text. In this paper, we explore the optimization of neural networks (NNs) for audio-based multimedia event classification , and discuss some insights...
Despite the significant progress made in the last years, state-of-the-art speech recognition technologies provide a satisfactory performance only in the close-talking condition. Robustness of distant speech recognition in adverse acoustic conditions, on the other hand, remains a crucial open issue for future applications of human-machine interactio...
This paper describes a new corpus of multi-channel audio data designed to study and develop distant-speech recognition systems able to cope with known interfering sounds propagating in an environment.
The corpus consists of both real and simulated signals and of a corresponding detailed annotation.
An extensive set of speech recognition experiments...
A smart lamp based on distant-talking speech recognition with a MEMS microphone array.
this video shows a prototype developed under the DIRHA project and currently working in a real apartment in Trento (Italy). It is a real-time, multi-room and multi-microphone distant-talking speech recognition system which allows the users to command and control with their voice most of the home appliances and devices. The system can work both with...
An example of the multi-microphone multi-room dataset generated under the EU DIRHA project. The target scenario is the domestic environment and the reference language is English
An example of the multi-microphone multi-room and multi-language database generated under the EU DIRHA project. The target scenario is the domestic environment with 40 sample-synchronized channels distributed over 5 different rooms of a real apartment are available.
This dataset can be used for distant-talking speech recognition experiments, multi-...
An example of the multi-microphone multi-room dataset generated under the EU DIRHA project. The target scenario is the domestic environment and the reference language is English.
This dataset can be used for distant-talking speech recognition experiments, multi-microphone signal processing, speaker localization, voice activity detection and many ot...
Distant-speech recognition represents a technology of fundamental importance for future development of assistive applications characterized by flexible and unobtrusive interaction in home environments. State-of-the-art speech recognition still exhibits lack of robustness, and an unacceptable performance variability, due to environmental noise, reve...