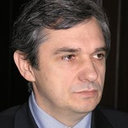
Ljubisa StankovicUniversity of Montenegro | UCG
Ljubisa Stankovic
Professor
Graph signal processing, Compressive sensing, Time-frequency analysis, Convolutional neural networks, Radar signals
About
450
Publications
102,716
Reads
How we measure 'reads'
A 'read' is counted each time someone views a publication summary (such as the title, abstract, and list of authors), clicks on a figure, or views or downloads the full-text. Learn more
9,976
Citations
Introduction
Additional affiliations
June 2013 - June 2014
Position
- Visiting academic
September 2003 - June 2008
January 2001 - March 2001
Publications
Publications (450)
Sparse signals can be recovered from a reduced set of samples by using
compressive sensing algorithms. The case when available samples are a random
subset of a uniformly or nonuniformly sampled signal is considered in this
paper. A recalculation procedure is used to reconstruct the nonuniformly
sampled signal. Signal recovery is done using an adapt...
The Ear-ECG provides a continuous Lead I like electrocardiogram (ECG) by measuring the potential difference related to heart activity by electrodes which are embedded within earphones. However, the significant increase in wearability and comfort enabled by Ear-ECG is often accompanied by a degradation in signal quality - a common obstacle that is s...
The matched filter is a crucial concept in both signal analysis and convolutional neural networks (CNNs). Previous work has addressed graph matched filtering principles for undirected graphs. This article expands upon the existing literature, by exploring matched filtering principles for signals on directed graphs. In such cases, the adjacency matr...
Directed acyclic graphs (DAGs) are used for modeling causal relationships, dependencies, and flows in various systems. However, spectral analysis becomes impractical in this setting because the eigen- decomposition of the adjacency matrix yields all eigenvalues equal to zero. This inherent property of DAGs results in an inability to differentiate b...
Synthetic aperture radar (SAR) automatic target recognition (ATR) is a crucial technique utilized in various scenarios of geoscience and remote sensing. Despite the remarkable success of convolutional neural networks (CNNs) in optical vision tasks, the application of CNNs in SAR ATR is still a challenging area due to the significant differences in...
Deep neural networks (DNNs) and especially convolutional neural networks (CNNs) have revolutionized the way we approach the analysis of large quantities of data. However, the largely ad hoc fashion of their development, albeit one reason for their rapid success, has also brought to light the intrinsic limitations of CNNs—in particular, those relate...
Generative adversarial networks (GANs) can synthesize abundant photo-realistic synthetic aperture radar (SAR) images. Some modified GANs (e.g., InfoGAN) are even able to edit specific properties of the synthesized images by introducing latent codes. It is crucial for SAR image synthesis since the targets in real SAR images have different properties...
A comprehensive approach to the spectrum characterization (derivation of eigenvalues and the corresponding multiplicities) for non-normalized, symmetric discrete trigonometric transforms (DTT) is presented in the paper. Eight types of the DTT are analyzed. New explicit analytic expressions for the eigenvalues, together with their multiplicities, fo...
Despite the tremendous success of convolutional neural networks (CNNs) in computer vision, the mechanism of CNNs still lacks clear interpretation. Currently, class activation mapping (CAM), a famous visualization technique to interpret CNN's decision, has drawn increasing attention. Gradient-based CAMs are efficient while the performance is heavily...
Forming the right combination of students in a group promises to enable a powerful and effective environment for learning and collaboration. However, defining a group of students is a complex task which has to satisfy multiple constraints. This work introduces an unsupervised algorithm for fair and skill-diverse student group formation. This is ach...
A class of doubly stochastic graph shift operators (GSO) is proposed, which is shown to exhibit: (i) lower and upper L2-boundedness for locally stationary random graph signals, (ii) L2-isometry for i.i.d. random graph signals with the asymptotic increase in the incoming neighbourhood size of vertices, and (iii) preservation of the mean of any graph...
The success of convolution neural networks (CNN) has been revolutionising the way we approach and use intelligent machines in the Big Data era. Despite success, CNNs have been consistently put under scrutiny owing to their \textit{black-box} nature, an \textit{ad hoc} manner of their construction, together with the lack of theoretical support and p...
Graph convolutional neural network (GCN) has drawn increasing attention and attained good performance in various computer vision tasks, however, there lacks a clear interpretation of GCN's inner mechanism. For standard convolutional neural networks (CNNs), class activation mapping (CAM) methods are commonly used to visualize the connection between...
Specific emitter identification (SEI) is the process of discriminating different emitters based on the radio fingerprints extracted from the received signals. Without complex parameter adjustment, radio fingerprints can be efficiently extracted by end-to-end deep learning models. However, deep learning models usually rely on a large number of train...
Image denoising is a vital image processing phase aiming to improve the quality of images and to make them more informative. In this paper, we propose a blind denoising approach for removing the outliers (impulsive disturbances) from digital images, by combining the random sample consensus (RANSAC) and compressive sensing (CS) principles. The propo...
Generative Adversarial Networks (GANs) can synthesize abundant photo-realistic synthetic aperture radar (SAR) images. Some recent GANs (e.g., InfoGAN), are even able to edit specific properties of the synthesized images by introducing latent codes. It is crucial for SAR image synthesis since the targets in real SAR images are with different propert...
Unit norm tight frames (UNTFs) provide redundant Parseval-like decompositions of vectors, and are viewed as the extensions of the orthonormal bases with more vector number than the vector dimension. UNTFs are known to be robust against additive noise and erasures, and have great potential as encoding schemes. However, the construction of UNTFs has...
This paper evaluates the performances of numerous encryption algorithms on mobile devices running the Android operating system. The primary objective of our research was to measure and compare the relative performances of tested algorithm implementations (Data Encryption Standard (DES), 3DES, Advanced Encryption Standard (AES), ChaCha20, Blowfish,...
Time-Frequency Signal Analysis and Processing (TFSAP) is a collection of theory and algorithms used for analysis and processing of nonstationary signals, as found in a wide range of applications including telecommunications, radar, and biomedical engineering. This book brings together the main knowledge of TFSAP, from theory to applications, in a u...
Convolutional neural networks (CNNs) have successfully achieved high accuracy in synthetic aperture radar (SAR) target recognition; however, the intransparency of CNNs is still a limiting or even disqualifying factor. Therefore, visually interpreting CNNs with SAR images has recently drawn increasing attention. Various class activation mapping (CAM...
The detection of the gravitational-wave (GW) events from the noisy measured data requires reliable filtering techniques. This paper provides the performance analysis of the intersection of confidence intervals (ICI) and local polynomial approximation (LPA) based techniques when applied to the filtering of the GW signals in the real-life, low signal...
Since compressive sensing deals with a signal reconstruction using a reduced set of measurements, the existence of a unique solution is of crucial importance. The most important approach to this problem is based on the restricted isometry property which is computationally unfeasible. The coherence index-based uniqueness criteria are computationally...
Deep Neural Networks (DNN) and especially Convolutional Neural Networks (CNN) are a de-facto standard for the analysis of large volumes of signals and images. Yet, their development and underlying principles have been largely performed in an ad-hoc and black box fashion. To help demystify CNNs, we revisit their operation from first principles and a...
Graph Convolutional Neural Networks (GCNN) are becoming a preferred model for data processing on irregular domains, yet their analysis and principles of operation are rarely examined due to the black box nature of NNs. To this end, we revisit the operation of GCNNs and show that their convolution layers effectively perform matched filtering of inpu...
In this paper, we present an approach to the reconstruction of signals exhibiting sparsity in a transformation domain, having some heavily disturbed samples. This sparsity-driven signal recovery exploits a carefully suited random sampling consensus (RANSAC) methodology for the selection of a subset of inlier samples. To this aim, two fundamental pr...
The paper presents an analysis and overview of vertex–frequency analysis, an emerging area in graph signal processing. A strong formal link of this area to classical time–frequency analysis is provided. Vertex–frequency localization-based approaches to analyzing signals on the graph emerged as a response to challenges of analysis of big data on irr...
Stock market returns are typically analyzed using standard regression, yet they reside on irregular domains which is a natural scenario for graph signal processing. To this end, we consider a market graph as an intuitive way to represent the relationships between financial assets. Traditional methods for estimating asset-return covariance operate u...
Within the compressive sensing (CS) paradigm, sparse signals can be reconstructed based on a reduced set of measurements, whereby reliability of the solution is determined by its uniqueness. With its mathematically tractable and feasible calculation, the coherence index is one of very few CS uniqueness metrics with considerable practical importance...
Since compressive sensing deals with a signal reconstruction using a reduced set of measurements, the existence of a unique solution is of crucial importance. The most important approach to this problem is based on the restricted isometry property which is computationally unfeasible. The coherence index-based uniqueness criteria are computationally...
Synthetic aperture radar (SAR) image interpretation has long been an important but challenging task in SAR imaging processing. Generally, SAR image interpretation comprises complex procedures including filtering, feature extraction, image segmentation, and target recognition, which greatly reduce the efficiency of data processing. In an era of deep...
Within the Compressive Sensing (CS) paradigm, sparse signals can be reconstructed based on a reduced set of measurements. Reliability of the solution is determined by the uniqueness condition. With its mathematically tractable and feasible calculation, coherence index is one of very few CS metrics with a considerable practical importance. In this p...
Electroencephalogram (EEG) signals are known to contain signatures of stimuli that induce brain activities. However, detecting these signatures to classify captured EEG waveforms is one of the most challenging tasks of EEG analysis. This paper proposes a novel time–frequency-based method for EEG analysis and characterization implemented in a comput...
A sparsity-assisted algorithm for the detection and removal of impulsive disturbances is presented. It is assumed that the uncorrupted signal is sparse or highly concentrated in the discrete cosine transform (DCT) domain or the windowed modified DCT (MDCT) domain. To detect whether a specific signal sample is corrupted by a disturbance, the gradien...
Classical portfolio optimization methods typically determine an optimal capital allocation through the implicit, yet critical, assumption of statistical time-invariance. Such models are inadequate for real-world markets as they employ standard time-averaging based estimators which suffer significant information loss if the market observables are no...
Digital images can be considered as sparse or approximately sparse in the two-dimensional discrete cosine transform domain. According to the compressive sensing theory, these images can be recovered from a reduced set of pixels. Such reconstructions are influenced by the noise and the non-reconstructed coefficients for approximately sparse images....
Graph signal processing deals with signals which are observed on an irregular graph domain. While many approaches have been developed in classical graph theory to cluster vertices and segment large graphs in a signal independent way, signal localization based approaches to the analysis of data on graph represent a new research direction which is al...
A class of multivariate spectral representations for real-valued nonstationary random variables is introduced, which is characterised by a general complex Gaussian distribution. In this way, the temporal signal properties -- harmonicity, wide-sense stationarity and cyclostationarity -- are designated respectively by the mean, Hermitian variance and...
The rapid growth in the amount of data in the digital world leads to the need for data compression, and so forth, reducing the number of bits needed to represent a text file, an image, audio, or video content. Compressing data saves storage capacity and speeds up data transmission. In this paper, we focus on the text compression and provide a compa...
The classical support uncertainty principle states that the signal and its discrete Fourier transform (DFT) cannot be localized simultaneously in an arbitrary small area in the time and the frequency domain. The product of the number of nonzero samples in the time domain and the frequency domain is greater or equal to the total number of signal sam...
The classical support uncertainty principle states that the signal and its discrete Fourier transform (DFT) cannot be localized simultaneously in an arbitrary small area in the time and the frequency domain. The product of the number of nonzero samples in the time domain and the frequency domain is greater or equal to the total number of signal sam...
Multichannel multicomponent signals can be decomposed into individual signal components by exploiting the eigendecomposition of the corresponding autocorrelation matrix. Recently, we have shown that such decomposition is possible even in the particularly challenging case of non-stationary components with significantly overlapped supports in their t...
In this paper, we present an approach to the reconstruction of signals exhibiting sparsity in a transformation domain, having some heavily disturbed samples. This sparsity-driven signal recovery exploits a carefully suited random sampling consensus (RANSAC) methodology for the selection of an inlier subset of samples. To this aim, two fundamental p...
The influence of finite-length registers and the corresponding quantization effects on the reconstruction of sparse and approximately sparse signals from a reduced set of measurements is analyzed in this paper. For the nonquantized measurements, the compressive sensing (CS) framework provides highly accurate reconstruction algorithms that produce n...
With its aim to reduce the amount of sensed data and to improve the energy efficiency, compressive sensing (CS) is recently witnessing a growing research interest in remote-sensing applications. The Fourier transform domain plays a significant role as a signal-processing tool and the sparsity domain for the CS-reconstruction methods. A generalized...
Many modern data analytics applications on graphs operate on domains where graph topology is not known a priori, and hence its determination becomes part of the problem definition, rather than serving as prior knowledge which aids the problem solution. Part III of this monograph starts by addressing ways to learn graph topology, from the case where...
Modern data analytics applications on graphs often operate on domains where graph topology is not known a priori, and hence its determination becomes part of the problem definition, rather than serving as prior knowledge which aids the problem solution. Part III of this monograph starts by a comprehensive account of ways to learn the pertinent grap...
The area of Data Analytics on graphs promises a paradigm shift, as we approach information processing of new classes of data which are typically acquired on irregular but structured domains (such as social networks, various ad-hoc sensor networks). Yet, despite the long history of Graph Theory, current approaches tend to focus on aspects of optimis...
Graphs are irregular structures that naturally represent the multifaceted data attributes; however, traditional approaches have been established outside signal processing and largely focus on analyzing the underlying graphs rather than signals on graphs. Given the rapidly increasing availability of multisensor and multinode measurements, likely rec...
A boundedness analysis is performed on a class of doubly stochastic graph shift operators (GSOs), which is shown to exhibit: (i) L1-isometry over graph shifts; (ii) lower and upper L2-norm boundedness with the asymptotic increase in the incoming neighbourhood size of vertices; and (iii) L2-isometry for i.i.d. graph signals. These properties are der...
Investment returns naturally reside on irregular domains, however, standard multivariate portfolio optimization methods are agnostic to data structure. To this end, we investigate ways for domain knowledge to be conveniently incorporated into the analysis, by means of graphs. Next, to relax the assumption of the completeness of graph topology and t...
The focus of Part I of this monograph has been on both the fundamental properties, graph topologies, and spectral representations of graphs. Part II embarks on these concepts to address the algorithmic and practical issues centered round data/signal processing on graphs, that is, the focus is on the analysis and estimation of both deterministic and...
A unitary shift operator (GSO) for signals on a graph is introduced, which exhibits the desired property of energy preservation over both backward and forward graph shifts. For rigour, the graph differential operator is also derived in an analytical form. The commutativity relation of the shift operator with the Fourier transform is next explored i...
With their ability to cater for simultaneously for multifaceted information, multichannel (multivariate) signals have been used to solve problems that are normally not solvable with signals obtained from a single source. One such problem is the decomposition of signals which comprise several components for which the domains of support significantly...
Graph signal processing deals with signals which are observed on an irregular graph domain. While many approaches have been developed in classical graph theory to cluster vertices and segment large graphs in a signal independent way, signal localization based approaches to the analysis of data on graph represent a new research direction which is al...
The area of Data Analytics on graphs promises a paradigm shift as we approach information processing of classes of data, which are typically acquired on irregular but structured domains (social networks, various ad-hoc sensor networks). Yet, despite its long history, current approaches mostly focus on the optimization of graphs themselves, rather t...
Influence of the finite-length registers and quantization effects on the reconstruction of sparse and approximately sparse signals is analyzed in this paper. For the nonquantized measurements, the compressive sensing (CS) framework provides highly accurate reconstruction algorithms that produce negligible errors when the reconstruction conditions a...
Binary compressive sensing (CS) is a relatively new idea in the theory of sparse signal reconstruction. Under this framework, the signal is reconstructed based on the sign of the available measurements. This paper analyzes basic one-bit CS concepts for the case of complex valued random Gaussian measurement matrices. The reconstruction is compared w...
A new form of the multivariate median is introduced. It is defined as a point in the multidimensional space whose sum of distances from a set of multidimensional hyperplanes is minimal. This median can be used to formulate and solve the problem of sparse signal reconstruction. Application of the proposed multivariate median is illustrated on exampl...
Analysis of vertex-varying spectral content of signals on graphs challenges the assumption of vertex invariance and requires the introduction of vertex-frequency representations as a new tool for graph signal analysis. Local smoothness, an important parameter of vertex-varying graph signals, is introduced and defined in this paper. Basic properties...
With their ability to handle an increased amount of information, multivariate and multichannel signals can be used to solve problems normally not solvable with signals obtained from a single source. One such problem is the decomposition signals with several components whose domains of support significantly overlap in both the time and the frequency...
Graphs are irregular structures which naturally account for data integrity, however, traditional approaches have been established outside Signal Processing, and largely focus on analyzing the underlying graphs rather than signals on graphs. Given the rapidly increasing availability of multisensor and multinode measurements, likely recorded on irreg...
The existence and uniqueness conditions are a prerequisite for reliable reconstruction of sparse signals from reduced sets of measurements within the Compressive Sensing (CS) paradigm. However, despite their underpinning role for practical applications, existing uniqueness relations are either computationally prohibitive to implement (Restricted Is...
Sparse signals are characterized by a few nonzero coefficients in one of their transformation domains. This was the main premise in designing signal compression algorithms. Compressive sensing as a new approach employs the sparsity property as a precondition for signal recovery. Sparse signals can be fully reconstructed from a reduced set of availa...
Graph signal processing deals with signals whose domain, defined by a graph, is irregular. An overview of basic graph forms and definitions is presented first. Spectral analysis of graphs is discussed next. Some simple forms of processing signal on graphs, like filtering in the vertex and spectral domain, subsampling and interpolation, are given. G...