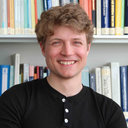
Kim Peter WabersichBosch · Department of Corporate Research
Kim Peter Wabersich
Dr. Sc.
About
40
Publications
7,416
Reads
How we measure 'reads'
A 'read' is counted each time someone views a publication summary (such as the title, abstract, and list of authors), clicks on a figure, or views or downloads the full-text. Learn more
1,345
Citations
Introduction
I’m a postdoctoral researcher in the intelligent control systems group at IDSC ETH. My research interests are in the fields of Safe learning, Model Predictive Control, Bayesian Optimization, and Autonomous Driving.
Publications
Publications (40)
Robotic systems typically have numerous parameters, e.g. the choice of planning algorithm, real-valued parameters of motion and vision modules, and control parameters. We consider the problem of optimizing these parameters for best worst-case performance over a range of environments. To this end we first propose to evaluate system parameters by adv...
The vision of autonomous driving is piecewise becoming reality. Still the problem of executing the driving task in a safe and comfortable way in all possible environments, for instance highway, city or rural road scenarios is a challenging task. In this paper we present a novel approach to planning trajectories for autonomous vehicles. Hereby we fo...
Today’s control engineering problems exhibit an unprecedented complexity, with examples including the reliable integration of renewable energy sources into power grids
[1]
, safe collaboration between humans and robotic systems
[2]
, and dependable control of medical devices
[3]
offering personalized treatment
[4]
. In addition to complianc...
We present an output feedback stochastic model predictive control (SMPC) approach for linear systems subject to Gaussian disturbances and measurement noise and probabilistic constraints on system states and inputs. The presented approach combines a linear Kalman filter for state estimation with an indirect feedback SMPC, which is initialized with a...
A predictive control barrier function (PCBF) based safety filter allows for verifying arbitrary control inputs with respect to future constraint satisfaction. The approach relies on the solution of two optimization problems computing the minimal constraint relaxations given the current state, and then computing the minimal deviation from a proposed...
This paper contrasts recursive state space models and direct multi-step predictors for linear predictive control. We provide a tutorial exposition for both model structures to solve the following problems: 1. stochastic optimal control; 2. system identification; 3. stochastic optimal control based on the estimated model. Throughout the paper, we pr...
This paper investigates the combination of model predictive control (MPC) concepts and posterior sampling techniques and proposes a simple constraint tightening technique to introduce cautiousness during explorative learning episodes. The provided theoretical analysis in terms of cumulative regret focuses on previously stated sufficient conditions...
While learning-based control techniques often outperform classical controller designs, safety requirements limit the acceptance of such methods in many applications. Recent developments address this issue through so-called predictive safety filters, which assess if a proposed learning-based control input can lead to constraint violations and modifi...
The growing need for high-performance controllers in safety-critical applications like autonomous driving motivated the development of formal safety verification techniques. In this letter, we design and implement a predictive safety filter that is able to maintain vehicle safety with respect to track boundaries when paired alongside any potentiall...
We propose an adaptive Model Predictive Safety Certification (MPSC) scheme for learning-based control of linear systems with bounded disturbances and uncertain parameters where the true parameters are contained within an a priori known set of parameters. An MPSC is a modular framework which can be used in combination with any learning-based control...
For control it is essential to obtain an accurate estimate of the current system state, based on uncertain sensor measurements and existing system knowledge. An optimization-based moving horizon estimation (MHE) approach uses a dynamical model of the system, and further allows to integrate physical constraints on system states and uncertainties, to...
The transfer of reinforcement learning (RL) techniques into real-world applications is challenged by safety requirements in the presence of physical limitations. Most RL methods, in particular the most popular algorithms, do not support explicit consideration of state and input constraints. In this paper, we address this problem for nonlinear syste...
While model predictive control (MPC) methods have proven their efficacy when applied to systems with safety specifications and physical limitations, their performance heavily relies on an accurate prediction model. As a consequence, a significant effort in the design of MPC controllers is dedicated to the modeling part and often requires advanced p...
In practical model predictive control (MPC) implementations, constraints on the states are typically softened to ensure feasibility despite unmodeled disturbances. In this work, we propose a soft constrained MPC formulation supporting polytopic terminal sets in half-space and vertex representation, which significantly increases the feasible set whi...
While learning-based control techniques often outperform classical controller designs, safety requirements limit the acceptance of such methods in many applications. Recent developments address this issue through so-called predictive safety filters, which assess if a proposed learning-based control input can lead to constraint violations and modifi...
Control, and in particular learning-based control, is challenging in large-scale and safety-critical system networks due to interactions between subsystems, which can potentially be time-varying. This paper presents a plug-and-play safety framework that can be applied together with high-performance control algorithms, e.g. emerging from learning te...
The growing need for high-performance controllers in safety-critical applications like autonomous driving has been motivating the development of formal safety verification techniques. In this paper, we design and implement a predictive safety filter that is able to maintain vehicle safety with respect to track boundaries when paired alongside any p...
Reinforcement learning (RL) methods have demonstrated their efficiency in simulation environments. However, many applications for which RL offers great potential, such as autonomous driving, are also safety critical and require a certified closed-loop behavior in order to meet safety specifications in the presence of physical constraints. This pape...
We present a stochastic model predictive control (MPC) method for linear discrete-time systems subject to possibly unbounded and correlated additive stochastic disturbance sequences. Chance constraints are treated in analogy to robust MPC using the concept of probabilistic reachable sets for constraint tightening. We introduce an initialization of...
Despite the success of reinforcement learning (RL) in various research fields, relatively few algorithms have been applied to industrial control applications. The reason for this unexplored potential is partly related to the significant required tuning effort, large numbers of required learning episodes, i.e. experiments, and the limited availabili...
Tight performance specifications in combination with operational constraints make model predictive control (MPC) the method of choice in various industries. As the performance of an MPC controller depends on a sufficiently accurate objective and prediction model of the process, a significant effort in the MPC design procedure is dedicated to modeli...
Recent successes in the field of machine learning, as well as the availability of increased sensing and computational capabilities in modern control systems, have led to a growing interest in learning and data-driven control techniques. Model predictive control (MPC), as the prime methodology for constrained control, offers a significant opportunit...
Distributed model predictive control methods for uncertain systems often suffer from considerable conservatism and can tolerate only small uncertainties, due to the use of robust formulations that are amenable to distributed design and computational methods. In this work, we propose a distributed stochastic model predictive control (DSMPC) scheme f...
While distributed algorithms provide advantages for the control of complex large-scale systems by requiring a lower local computational load and less local memory, it is a challenging task to design high-performance distributed control policies. Learning-based control algorithms offer promising opportunities to address this challenge, but generally...
While distributed algorithms provide advantages for the control of complex large-scale systems by requiring a lower local computational load and less local memory, it is a challenging task to design high-performance distributed control policies. Learning-based control algorithms offer promising opportunities to address this challenge, but generally...
Reinforcement learning (RL) methods have demonstrated their efficiency in simulation environments. However, many applications for which RL offers great potential, such as autonomous driving, are also safety critical and require a certified closed-loop behavior in order to meet safety specifications in the presence of physical constraints. This pape...
We present a stochastic model predictive control (MPC) method for linear discrete-time systems subject to possibly unbounded and correlated additive stochastic disturbance sequences. Chance constraints are treated in analogy to robust MPC using the concept of probabilistic reachable sets for constraint tightening. We introduce an initialization of...
Despite fast progress in Reinforcement Learning (RL), the transfer into real-world applications is challenged by safety requirements in the presence of physical limitations. This is often due to the fact, that most RL methods do not support explicit consideration of state and input constraints. In this paper, we address this problem for nonlinear s...
We present an economic model predictive control scheme for general nonlinear systems based on a terminal cost and a terminal constraint set. We study in particular systems which are optimally operated at some periodic orbit. Besides recursive feasibility of the control scheme, we provide an asymptotic average performance bound which is no worse tha...
Dynamical systems with stochastic uncertainties are ubiquitous in the field of control, with linear systems under additive Gaussian disturbances a most prominent example. The concept of probabilistic invariance was introduced to extend the widely applied concept of invariance to this class of problems. Computational methods for their synthesis, how...
While it has been repeatedly shown that learning-based controllers can provide superior performance, they often lack of safety guarantees. This paper aims at addressing this problem by introducing a model predictive safety certification (MPSC) scheme for polytopic linear systems with additive disturbances. The scheme verifies safety of a proposed l...
The control of complex systems faces a trade-off between high performance and safety guarantees, which in particular restricts the application of learning-based methods to safety-critical systems. A recently proposed framework to address this issue is the use of a safety controller, which guarantees to keep the system within a safe region of the st...
Bayesian Optimization (BO) has become a core method for solving expensive black-box optimization problems. While much research focussed on the choice of the acquisition function, we focus on online length-scale adaption and the choice of kernel function. Instead of choosing hyperparameters in view of maximum likelihood on past data, we propose to u...