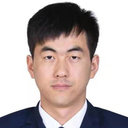
Kai ChengTechnische Universität München | TUM · Chair of Engineering Risk Analysis
Kai Cheng
Doctor of Philosophy
About
45
Publications
11,710
Reads
How we measure 'reads'
A 'read' is counted each time someone views a publication summary (such as the title, abstract, and list of authors), clicks on a figure, or views or downloads the full-text. Learn more
1,237
Citations
Introduction
Uncertainty quantification, Surrogate model, Model reduction.
Education
March 2018 - September 2021
September 2015 - February 2018
Publications
Publications (45)
In the context of uncertainty analysis, Polynomial chaos expansion (PCE) has been proven to be a powerful tool for developing meta-models in a wide range of applications, especially for sensitivity analysis. But the computational cost of classic PCE grows exponentially with the size of the input variables. An efficient approach to address this prob...
An established way to tackle model nonlinearities in projection-based model reduction is
via relying on partial information. This idea is shared by the methods of
gappy proper orthogonal decomposition (POD), missing point estimation (MPE), masked projection,
hyper reduction and the (discrete) empirical interpolation method (DEIM).
The selected in...
In this paper, we propose a sequential directional importance sampling (SDIS) method for rare event estimation. SDIS expresses a small failure probability in terms of a sequence of auxiliary failure probabilities, defined by magnifying the input variability. The first probability in the sequence is estimated with Monte Carlo simulation in Cartesian...
In this paper, a novel gradient-enhanced (GE) polynomial chaos expansion (PCE) model is proposed for approximating complex computational models. We start from the assumption that the PCE model and its partial derivatives with respect to each of the input parameters are Gaussian processes and assign a Gaussian prior to the unknown coefficients. Then...
Gradient-enhanced Kriging (GE-Kriging) is a well-established surrogate modelling technique for approximating expensive computational models. However, it tends to get impractical for high-dimensional problems due to the size of the inherent correlation matrix and the associated high-dimensional hyper-parameter tuning problem. To address these issues...
This paper proposes a generalized subset simulation (GSS) method for estimating small failure probability. The basic idea is to modify the failure threshold and amplify the variability of input variables simultaneously in a sequential way. With a proper choice of the intermediate failure thresholds and amplification factors, the problem of estimati...
In this paper, we propose a sequential directional importance sampling (SDIS) method for rare event estimation. SDIS expresses a small failure probability in terms of a sequence of auxiliary failure probabilities, defined by magnifying the input variability. The first probability in the sequence is estimated with Monte Carlo simulation in Cartesian...
In the study, we propose a new polynomial chaos expansion surrogate modeling method based on Adaboost (Adaboost-PCE) for uncertainty quantification. Adaboost is an ensemble learning technique originating from the machine learning field. The idea of Adaboost-PCE is to construct many times the “weak” PCE model with assigned weights to each sample poi...
In structural reliability analysis, the estimation of rare failure probability is a significant challenge. Directional importance sampling may address this challenge when a quasi-optimal sampling density is chosen. Directional importance sampling involves sampling on the unit hypersphere and one-dimensional reliability analysis. This paper develops...
Reliability-based design optimization (RBDO) aims at minimizing general cost under the reliability constraints by considering the inherent uncertainties in engineering. In this work, we develop a decoupled RBDO approach named RIFA-ADK which aims at reliability index function (RIF) approximation by adaptive double-loop Kriging. The proposed RIFA-ADK...
In this paper, a novel reliability method called AK-DS for adaptive Kriging (AK)-based directional sampling (DS) is proposed to efficiently estimate small failure probability. The AK-MCS method, a popular reliability method, associates adaptive Kriging with Monte Carlo Simulation to minimize the performance function evaluations. For small failure p...
In this paper, a fully decoupled simulation method is proposed for reliability-based design optimization (RBDO) based on thermodynamic integration and parallel tempering (TIPT). We show that the failure probability function and its gradient can be obtained simultaneously with once generalized reliability analysis, and thus the RBDO problem is conve...
In this paper, Bayesian support vector regression (SVR) model is developed for structural reliability analysis adaptively. Two SVR models, namely, least-square SVR and ε-SVR, are constructed under the Bayesian inference framework with a square loss function and a ε-insensitive square one respectively. In this framework, a Gaussian process prior is...
Machine learning techniques have received much attention in many areas for regression and classification tasks. In this paper, two new support vector regression (SVR) models, namely, least-square SVR and e-SVR, are developed under the Bayesian inference framework with a square loss function and a e-insensitive squared one respectively. In this fram...
Global sensitivity analysis has mainly been analyzed for scalar output and static models, though many mathematical and computational models used in engineering produce multivariate output that show some degree of correlation, and most physical systems are dynamic models. This paper focuses on global sensitivity analysis for multivariate output and...
Assessing the failure probability of complex aeronautical structure is a difficult task in presence of uncertainties. In this paper, active learning polynomial chaos expansion (PCE) is developed for reliability analysis. The proposed method firstly assigns a Gaussian Process (GP) prior to the model response, and the covariance function of this GP i...
Surrogate models are popular tool to approximate the functional relationship of expensive simulation models in multiple scientific and engineering disciplines. Successful use of surrogate models can provide significant savings of computational cost. However, with a variety of surrogate model approaches available in literature, it is a difficult tas...
Local reliability sensitivity and global reliability sensitivity are required in reliability-based design optimization, since they can provide rich information including variable importance ranking and gradient information. However, traditional Monte Carlo simulation is inefficient for engineering application. A novel numerical simulation method ba...
Assessing the failure probability of complex structure is a difficult task in presence of various uncertainties. In this paper, a new adaptive approach is developed for reliability analysis by ensemble learning of multiple competitive surrogate models, including Kriging, polynomial chaos expansion and support vector regression. Ensemble of surrogat...
In this paper, hierarchical surrogate model combined with dimensionality reduction technique is investigated for uncertainty propagation of high‐dimensional problems. In the proposed method, a low‐fidelity sparse polynomial chaos expansion model is firstly constructed to capture the global trend of model response and exploit a low‐dimensional activ...
Polynomial chaos expansion (PCE) has been proven to be a powerful tool for developing surrogate models in various engineering fields for uncertainty quantification. The computational cost of full PCE is unaffordable due to the “curse of dimensionality” of the expansion coefficients. In this paper, an expanded sparse Bayesian learning method for spa...
Sparse polynomial chaos expansion has been widely used to tackle problems of function approximation in the field of uncertain quantification. The accuracy of PCE depends on how to construct the experimental design. Therefore, adaptive sampling methods of designs of experiment are raised. Classic designs of experiment for PCE are based on least-squa...
Time-variant reliability analysis aims at estimating the probability that an engineering system successfully performs intended missions over a certain period of time under various sources of uncertainty. In order to perform the time-variant reliability analysis efficiently, this paper presents a high dimensional model representation (HDMR) model co...
Recently, gradient-enhanced surrogate models have drawn extensive attention for function approximation, in which the gradient information is utilized to improve the surrogate model accuracy. In this work, gradient-enhanced high dimensional model representation (HDMR) is established based on Bayesian inference technique. The proposed method first as...
Surrogate modeling techniques have been increasingly developed for optimization and uncertainty quantification problems in many engineering fields. The development of surrogates requires modeling high dimensional and non‐smooth functions with limited information. To this end, the hybrid surrogate modeling method, where different surrogate models ar...
Models with multivariate outputs are widely used for risk assessment and decision-making in practical applications. In this paper, multi-output support vector regression (M-SVR) is employed for global sensitivity analysis (GSA) with multivariate output models. The orthogonal polynomial kernel is used to build the M-SVR meta-model, and the covarianc...
Global reliability sensitivity index, defined as the average absolute difference between the original probability density function (PDF) of input and the conditional one on the failure event, can measure the effect of input on the failure probability and provide information for the safety design. However, efficiently estimating the global reliabili...
Global sensitivity analysis, such as Sobol’ indices, plays an important role for quantifying the relative importance of random inputs to the response of complex model, and the estimation of Sobol’ indices is a challenging problem. In this paper, Bayesian Monte Carlo method is employed for developing a new technique to estimate the Sobol’ indices wi...
Quantile regression has emerged as a significant extension of traditional linear models, and its appealing features, such as robustness, efficiency in the presence of censoring and flexibility of modeling stress-life relationship, have recently been recognized for analyzing accelerated life test data. Based on these merits, we present a method for...
Polynomial chaos expansion (PCE) has been proven to be a powerful tool for developing surrogate models in the field of uncertainty and global sensitivity analysis. The computational cost of classical PCE is unaffordable since the number of terms grows exponentially with the dimensionality of inputs. This considerably restricts the practical use of...
Competing risk usually exists in engineering applications; thus, the study of the statistical inference of accelerated life testing with competing failure modes is of great significance. In this article, we address the statistical analysis of a step-stress accelerated life test in the presence of dependent competing failure modes. The dependence st...
The moment-independent importance measure technique for exploring how uncertainty allocates from output to inputs has been widely used to help engineers estimate the degree of confidence of decision results and assess risks. Solving the Borgonovo moment-independent importance measure in the presence of the multivariate output is still a challenging...
Polynomial chaos expansion (PCE) is widely used by engineers and modelers in various engineering fields for uncertainty analysis. The computational cost of full PCE is unaffordable for the “curse of dimensionality” of the expansion coefficients. In this paper, a new method for developing sparse PCE is proposed based on the diffeomorphic modulation...
Aiming at efficiently estimating the dynamic failure probability with multiple temporal and spatial parameters and analyzing the global reliability sensitivity of the dynamic problem, a method is presented on the moment estimation of the extreme value of the dynamic limit state function. Firstly, two strategies are proposed to estimate the dynamic...
In accelerated life tests (ALT), there are many limitations for a traditional parametric method to estimate a particular quantile in the lower tail of the lifetime distribution at the use condition. This article develops a Bayesian quantile regression method for analyzing ALT data. As a distribution-free method, Bayesian quantile regression method...