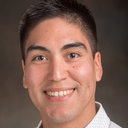
Juan M. CardenasUniversity of Colorado Boulder | CUB · Department of Aerospace Engineering Sciences (AES)
Juan M. Cardenas
Ph. D. in Applied Mathematics
About
11
Publications
568
Reads
How we measure 'reads'
A 'read' is counted each time someone views a publication summary (such as the title, abstract, and list of authors), clicks on a figure, or views or downloads the full-text. Learn more
32
Citations
Introduction
My research interest lies in the intersection of applied mathematics, scientific computing, and machine learning. The motivations for my research are surrogate model construction in Uncertainty Quantification (UQ), data-driven learning problems, medical imaging, approximation theory, and compressed sensing.
Skills and Expertise
Publications
Publications (11)
We introduce a general framework for active learning in regression problems. Our framework extends the standard setup by allowing for general types of data, rather than merely pointwise samples of the target function. This generalization covers many cases of practical interest, such as data acquired in transform domains (e.g., Fourier data), vector...
This work analyses a high-order hybridizable discontinuous Galerkin (HDG) method for the linear elasticity problem in a domain not necessarily polyhedral. The domain is approximated by a polyhedral computational domain where the HDG solution can be computed. The introduction of the rotation as one of the unknowns allows us to use the gradient of th...
The problem of approximating smooth, multivariate functions from sample points arises in many applications in scientific computing, e.g., in computational Uncertainty Quantification (UQ) for science and engineering. In these applications, the target function may represent a desired quantity of interest of a parameterized Partial Differential Equati...
The problem of approximating smooth, multivariate functions from sample points arises in many applications in scientific computing, e.g., in computational Uncertainty Quantification (UQ) for science and engineering. In these applications, the target function may represent a desired quantity of interest of a parameterized Partial Differential Equati...
In this chapter, we discuss recent work on learning sparse approximations to high-dimensional functions on data, where the target functions may be scalar-,’ vector- or even Hilbert space-valued. Our main objective is to study how the sampling strategy affects the sample complexity—that is, the number of samples that suffice for accurate and stable...
This work analyzes a high order hybridizable discontinuous Galerkin (HDG) method for the linear elasticity problem in a domain not necessarily polyhedral. The domain is approximated by a polyhedral computational domain where the HDG solution can be computed. The introduction of the rotation as one of the unknowns allows us to use the gradient of th...
In this chapter, we discuss recent work on learning sparse approximations to high-dimensional functions on data, where the target functions may be scalar-, vector- or even Hilbert space-valued. Our main objective is to study how the sampling strategy affects the sample complexity -- that is, the number of samples that suffice for accurate and stabl...
Many problems in computational science and engineering can be described in terms of approximating a smooth function of $d$ variables, defined over an unknown domain of interest $\Omega\subset \mathbb{R}^d$, from sample data. Here both the curse of dimensionality ($d\gg 1$) and the lack of domain knowledge with $\Omega$ potentially irregular and/or...
In this paper, we address the problem of approximating a multivariate function defined on a general domain in $d$ dimensions from sample points. We consider weighted least-squares approximation in an arbitrary finite-dimensional space $P$ from independent random samples taken according to a suitable measure. In general, least-squares approximations...