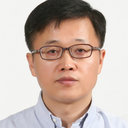
Jongmyon KimUniversity of Ulsan | UOU · School of IT convergence
Jongmyon Kim
PhD
About
505
Publications
196,303
Reads
How we measure 'reads'
A 'read' is counted each time someone views a publication summary (such as the title, abstract, and list of authors), clicks on a figure, or views or downloads the full-text. Learn more
7,512
Citations
Introduction
Skills and Expertise
Additional affiliations
March 2007 - July 2017
Publications
Publications (505)
Fault diagnosis of gears plays an important role in reducing downtime and maximizing efficiency of rotating machinery. Vibration is popular parameter for gear fault detection. The occurrence of faults produces recurring transient impulses in the gear vibration signals. However, these transient features are heavily masked by background noises making...
The prediction of the remaining useful life (RUL) is important for the conditions of rotating machinery to maintain reliability and decrease losses. This study proposes an efficient approach based on an adaptive maximum second-order cyclostationarity blind deconvolution (ACYCBD) and a convolutional LSTM autoencoder to achieve the feature extraction...
This study introduces an innovative approach for fault diagnosis of a multistage centrifugal pump (MCP) using explanatory ratio (ER) linear discriminant analysis (LDA). Initially, the method addresses the challenge of background noise and interference in vibration signals by identifying a fault-sensitive frequency band (FSFB). From the FSFB, raw hy...
This paper proposes a fault diagnosis method for centrifugal pumps (CP) based on multi-filter processed scalograms (MFS) and convolutional neural networks (CNN). Deep learning (DL) based autonomous Health-sensitive features extraction from continuous wavelet transform (CWT) scalograms are popular adoption for the health diagnosis of centrifugal pum...
This paper proposes a new fault diagnosis method for centrifugal pumps by combining signal processing with deep learning techniques. Centrifugal pumps facilitate fluid transport through the energy generated by the impeller. Throughout the operation, variations in the fluid pressure at the pump’s inlet may impact the generalization of traditional ma...
To prevent potential instability the early detection of cracks is imperative due to the prevalent use of concrete in critical infrastructure. Automated techniques leveraging artificial intelligence, machine learning, and deep learning as the traditional manual inspection methods are time-consuming. The existing automated concrete crack detection al...
This paper proposes a novel approach to predicting the useful life of rotating machinery and making fault diagnoses using an optimal blind deconvolution and hybrid invertible neural network. First, a new optimal adaptive maximum second-order cyclostationarity blind deconvolution (OACYCBD) is developed for denoising vibration signals obtained from r...
Pipelines hold a crucial role in the effective transportation of liquids and gases over extensive distances. Detecting leaks through Acoustic Emission (AE) events is intricate due to the interference of noise. The conventional strategy of utilizing AE scalogram images from continuous wavelet transforms (CWT) faces challenges in precisely identifyin...
Centrifugal pump fault detection and isolation in an early stage is essential from an industry and consumer viewpoint for significantly reducing downtime and maintenance activities., resulting in cost savings. This paper presents a comprehensive framework for centrifugal pump fault diagnosis integrating wavelet coherence analysis and hybrid classif...
This paper proposes a new method for bearing fault diagnosis using wavelet packet transform (WPT)-based signal representation and informative factor linear discriminant analysis (IF-LDA). Time–frequency domain approaches for analyzing bearing vibration signals have gained wide acceptance due to their effectiveness in extracting information related...
This paper proposes a novel and reliable leak-detection method for pipeline systems based on acoustic emission (AE) signals. The proposed method analyzes signals from two AE sensors installed on the pipeline to detect leaks located between these two sensors. Firstly, the raw AE signals are preprocessed using empirical mode decomposition. The time d...
In this paper, an approach to perform leak state detection and size identification for industrial fluid pipelines with an acoustic emission (AE) activity intensity index curve (AIIC), using b-value and a random forest (RF), is proposed. Initially, the b-value was calculated from pre-processed AE data, which was then utilized to construct AIICs. The...
This work presents a technique for fault detection and identification in centrifugal pumps (CPs) using a novel fault-specific Mann–Whitney test (FSU Test) and K-nearest neighbor (KNN) classification algorithm. Traditional fault indicators, such as the mean, peak, root mean square, and impulse factor, lack sensitivity in detecting incipient faults....
A new framework for the fault diagnosis of centrifugal pumps (CP) is presented in this paper. Time domain (TD) features obtained from the vibration signal (VS) of the CP are vulnerable to severe faults and can affect the fault classification accuracy of the classifier. To address this issue, the proposed method selects a healthy reference signal (H...
Wavelet packet transform (WPT) has found extensive use in bearing fault diagnosis for its ability to provide more accurate frequency and time-frequency representations of non-stationary signals. Traditional quantitative methods prioritize unequal node-energy distribution at the desired decomposition level as a criterion for WPT base selection. Deco...
This paper proposes an intelligent framework for the fault diagnosis of centrifugal pumps (CPs) based on wavelet coherence analysis (WCA) and deep learning (DL). The fault-related impulses in the CP vibration signal are often attenuated due to the background interference noises, thus affecting the sensitivity of the traditional statistical features...
This paper proposes a new framework for leak diagnosis in pipelines using leak-augmented scalograms and deep learning. Acoustic emission (AE) scalogram images obtained from the continuous wavelet transform have been useful for pipeline health diagnosis, particularly when combined with deep learning. However, background noise has a significant impac...
This paper presents a novel framework for classifying ongoing conditions in centrifugal pumps based on signal processing and deep learning techniques. First, vibration signals are acquired from the centrifugal pump. The acquired vibration signals are heavily affected by macrostructural vibration noise. To overcome the influence of noise, pre-proces...
The 4.0 industry revolution and the prevailing technological advancements have made industrial units more intricate. These complex electro-mechanical units now aim to improve efficiency and increase reliability. Downtime of such essential units in the current competitive age is unaffordable. The paradigm of fault diagnostics is being shifted from c...
This paper proposes a novel technique for leak detection and localization in industrial fluid
pipelines. Artificial intelligence-based supervised methods are a popular adoption for pipeline
leak identification. However, supervised techniques need prior knowledge about pipeline failure
for training purposes. To address this challenge, a new method f...
This paper proposes a leak detection and size identification technique in fluid pipelines based on a new leak-sensitive feature called the vulnerability index (VI) and 1-D convolutional neural network (1D-CNN). The acoustic emission hit (AEH) features can differentiate between normal and leak operating conditions of the pipeline. However, the multi...
Bearings are critical components of motors. However, they can cause several issues. Proper and timely detection of faults in the bearings can play a decisive role in reducing damage to the entire system, thereby reducing economic losses. In this study, a hybrid fuzzy V-structure fuzzy fault estimator was used for fault diagnosis and crack size iden...
In this study, a scheme for leak localization on a cylinder tank bottom using acoustic emission (AE) is proposed. This approach provides a means of early failure detection, thus reducing financial damage and hazards to the environment and users. The scheme starts with the hit detection process using a constant false alarm rate (CFAR) and a fixed th...
A framework aimed to improve the bearing-fault diagnosis accuracy using a hybrid fea-ture-selection method based on Wrapper-WPT is proposed in this paper. In the first step, the enve-lope vibration signal of the roller bearing is provided to the Wrapper-WPT. There, it is initially de-composed into several sub-bands using Wavelet Packet Transform (W...
Rolling bearings are treated as important machinery power components, faults of rolling bearings affect machinery operation, so an intelligent fault diagnosis method is very useful of safety operation in rolling bearings. This paper proposes a novel fault diagnosis method based on improved Adaptive Deep Convolution Neural Networks algorithm to real...
Condition monitoring becomes an integral part of the industrial manufacturing system to ensure a safe working environment and reduce the cost of maintenance. Involving deep learning techniques in fault diagnosis methods not only increases the accuracy and reliability of the system but also reduces the operation time and hassle of the manual feature...
In the machine learning and data science pipelines, feature extraction is considered the most crucial component according to researchers, where generating a discriminative feature matrix is the utmost challenging task to achieve high classification accuracy. Generally, the classical feature extraction techniques are sensitive to the noisy component...
SI: The Role of Data Science, and Computer Vision in Public Health
The use of massive amounts of data has become critical in
the public health sector. We have seen several cases
throughout this COVID-19 outbreak. We have witnessed
significant advancements in cancer detection, COVID x-ray
processing, brain signal analysis, and stress analysis using...
Rotating machinery plays an important role in industrial systems, and faults in the machinery may damage the system health. A novel image-based diagnosis method using improved deep convolutional generative adversarial networks (DCGAN) is proposed for the feature recognition and fault classification of rotating machinery. First, vibration signal dat...
A gearbox is a critical rotating component that is used to transmit torque from one shaft to another. This paper presents a data-driven gearbox fault diagnosis system in which the issue of variable working conditions namely uneven speed and the load of the machinery is addressed. Moreover, a mechanism is suggested that how an improved feature extra...
A novel intelligent centrifugal pump (CP) fault diagnosis method is proposed in this paper. The method is based on the contrast in vibration data obtained from a centrifugal pump (CP) under several operating conditions. The vibration signals data obtained from a CP are non-stationary because of the impulses caused by different faults; thus, traditi...
Active acoustic emission (AE) signal estimation is crucial for realizing high-precision bearing fault diagnosis. However, the identification of the bearing fault in the low-speed motor is still a challenging issue. In this article, observer-based low-speed bearing fault identification is investigated, and an observer with adaptive fuzzy switching g...
Bearings cause the most breakdowns in induction motors, which can result in significant economic losses. If faults in the bearings are not detected in time, they can cause the whole system to fail. System failures can lead to unexpected breakdowns, threats to worker safety, and huge economic losses. In this investigation, a new approach is proposed...
Diagnostics of mechanical problems in manufacturing systems are essential to maintaining safety and minimizing expenditures. In this study, an intelligent fault classification model that combines a signal-to-image encoding technique and a convolution neural network (CNN) with the motor-current signal is proposed to classify bearing faults. In the b...
About this Research Topic
In recent years, energy industries have been facing increasing pressure on the immediate hike in energy demands and environmental protection. The research on “Smart Grid” and “Renewable Energy” has been mainstreamed to cope with this grand challenge. Smart grid technology is enabling an effective distribution and managemen...
Using an adaptive noise canceling technique (ANCT) and distance ratio principal component analysis (DRPCA), this paper proposes a new fault diagnostic model for multi-degree tooth-cut failures (MTCF) in a gearbox operating at inconsistent speeds. To account for background and disturbance noise in the vibration characteristics of gear failures, the...
Acoustic emission techniques are widely used to monitor industrial pipelines. Intelligent methods using acoustic emission signals can analyze acoustic waves and provide important information for leak detection and localization. To address safety and protect the operation of industrial pipelines, a novel hybrid approach based on acoustic emission si...
This paper proposes a new technique for the construction of a concrete-beam health indicator based on the Kullback–Leibler divergence (KLD) and deep learning. Health indicator (HI) construction is a vital part of remaining useful lifetime (RUL) approaches for monitoring the health of concrete structures. Through the construction of a HI, the deteri...
Deep learning techniques are gaining popularity due to their ability of feature extraction, dimensionality reduction, and classification. However, one of the biggest challenges in bearing fault diagnosis is reliable feature extraction. When using the bearing fault vibration spectrum, the deep neural network (DNN) model can learn the relationships i...
A rub-impact fault is recognized as a complex, non-stationary, and non-linear type of mechanical fault that frequently occurs in turbines. Extracting features for diagnosing rubbing faults at their early stages requires complex and computationally expensive signal processing approaches that are not always suitable for industrial applications. Furth...
Bearings are used to reduce inertia in numerous utilizations. Lately, anomaly detection and identification in the bearing using acoustic emission signals has received attention. In this work, the combination of the machine learning and adaptive-backstepping digital twin approach is recommended for bearing anomaly size identification. The proposed a...
Condition monitoring can avoid sudden breakdown and ensure the reliable and safe operation of rotating machinery used in the industry. The early detection of fault signatures and accurately classifying them in time will ensure efficient maintenance operation and reduce the possibility of losses due to uncertain breakdown. In the fault diagnosis mec...
This paper proposes a reliable technique for pipeline leak detection using acoustic emission signals. The acoustic emission signal of a pipeline contains leak-related information. However, the noise in the signal often obscures the leak-related information, making traditional acoustic emission features, such as count and peaks, less effective. To o...
Bearings are nonlinear systems that can be used in several industrial applications. In this study, the combination of a strict-feedback backstepping digital twin and machine learning algorithm was developed for bearing crack type/size diagnosis. Acoustic emission sensors were used to collect normal and abnormal data for various crack sizes and moto...
One of the most critical assignments in fault diagnosis is to decide the finest set of features by evaluating the statistical parameters of the time-domain signals. However, these parameters are vulnerable under variable speed conditions, i.e., different loads, and speeds to capture the dynamic attributes of various health types. Therefore, this pa...
This paper proposes a new fault diagnosis framework for Centrifugal Pump (CP) fault diagnosis. To utilize the fault-related transients, the proposed fault diagnosis framework first preprocesses the vibration signal (VS) using wavelet packet transform (WPT). Instead of extracting features from a specific wavelet packet transform base (node), the pro...
Bearing fault diagnosis is essential in manufacturing systems to avoid problems such as downtime costs. Convolutional neural network (CNN) models have enabled a new generation of intelligent bearing fault diagnosis methods for smart manufacturing owing to their capability to extract features for 2-dimensional (2D) representations, such as signals r...
The EEG signal is an electrical flow between brain neurons, and it appears differently depending on the mental and physical state. In this paper, stress is classified by analyzing EEG signals based on artificial intelligence. In this paper, using the DEAP dataset the stress state and the non-stress state were separated and trained in an artificial...
Existing acoustic emission signal–based methods for pipeline leak detection usually denoise the raw signals directly in signal–space, then extract signatures from denoised signals, and finally classify normal/leaky states via classifiers trained using offline datasets. Their complex computational structures may limit their real–time application, es...
Dear Colleagues,
Machinery and mechanical structures in the industry suffer from inevitable degradation and performance degradation during operation. By collecting and processing data using a variety of sensors, timely diagnosis of symptoms of deterioration and reliable estimation of future health conditions are essential for industrial productivi...
This study proposes a fault diagnosis method (FD) for multistage centrifugal pumps (MCP) using informative ratio principal component analysis (Ir-PCA). To overcome the interference and background noise in the vibration signatures (VS) of the centrifugal pump, the fault diagnosis method selects the fault-specific frequency band (FSFB) in the first s...
Statistical features extraction from bearing fault signals requires a substantial level of knowledge and domain expertise. Furthermore, existing feature extraction techniques are mostly confined to selective feature extraction methods namely, time-domain, frequency-domain, or time-frequency domain statistical parameters. Vibration signals of bearin...
Fault diagnosis and classification for machines are integral to condition monitoring in the industrial sector. However, in recent times, as sensor technology and artificial intelligence have developed, data-driven fault diagnosis and classification have been more widely investigated. The data-driven approach requires good-quality features to attain...
Pipelines are used to transport liquids and gases
between different places. In recent years, identifying the
location and size of cracks in transmission pipes has received
much attention. Thus, in this research, the combination of fuzzy
digital twin (FDT), support vector machine (SVM), and
backstepping (BS) observer is suggested for leak detec...
Pipeline leakage remains a challenge in various industries. Acoustic emission (AE) technology has recently shown great potential for leak diagnosis. Many AE features, such as root mean square (RMS), peak value, standard deviation, mean value, and entropy, have been suggested to detect leaks. However, background noise in AE signals makes these featu...
Breast cancer is now the most frequently diagnosed cancer in women, and its percentage is gradually increasing. Optimistically, there is a good chance of recovery from breast cancer if identified and treated at an early stage. Therefore, several researchers have established deep-learning-based automated methods for their efficiency and accuracy in...
In this study, a scheme of remaining useful lifetime (RUL) prognosis from raw acoustic emission (AE) data is presented to predict the concrete structure's failure before its occurrence, thus possibly prolong its service life and minimizing the risk of accidental damage. The deterioration process is portrayed by the health indicator (HI), which is a...
A rub-impact fault is a complex, nonstationary, and nonlinear fault that occurs in turbines. Extracting features for diagnosing rubbing faults at their early stages requires complex and computationally expensive signal processing approaches that are not always suitable for industrial applications. In this article, a hybrid approach that uses a comb...
In this paper, we propose a three-stage lightweight framework for centrifugal pump fault diagnosis. First, the centrifugal pump vibration signatures are fast transformed using a Walsh transform, and Walsh spectra are obtained. To overcome the hefty noise produced by macro-structural vibration, the proposed method selects the fault characteristic co...
This paper presents a data-driven prognostic framework for rolling-element bearings (REBs). This framework infers a bearing’s health index by defining a degree-of-defectiveness (DD) metric in the frequency domain of bearing raw signal, named DD-based health index (DDHI). Then, we systematically apply least-square support vector machines (LSSVMs) in...
Deep learning (DL) plays a very important role in the fault diagnosis of rotating machinery. To enhance the self-learning capacity and improve the intelligent diagnosis accuracy of DL for rotating machinery, a novel hybrid deep learning method (NHDLM) based on Extended Deep Convolutional Neural Networks with Wide First-layer Kernels (EWDCNN) and lo...
This paper proposes a Gaussian mixture model-based (GMM) bearing fault band selection (GMM-WBBS) method for signal processing. The proposed method benefits reliable feature extraction using fault frequency oriented Gaussian mixture model (GMM) window series. Selecting exclusively bearing fault frequency harmonics, it eliminates the interference of...
Bearing failure is considered as one of the major problems in induction motor, which can result a huge mechanical damage if is not monitored from the initial stage. A complete fault classification method is presented in this paper by combining wavelet-based signal processing technique and deep learning method for fault classification. Vibration sig...
Bearings prevent damage caused by frictional forces between parts supporting the rotation and they keep rotating shafts in their correct position. However, the continuity of work under harsh conditions leads to inevitable bearing failure. Thus, methods for bearing fault diagnosis (FD) that can predict and categorize fault type, as well as the level...
Induction motors are consumed around 80% of energy in heavy industries, that approximately 20% of this energy consumption is because of mechanical failures. Moreover, the bearing failure with about 69% is the principal constituent of mechanical defects. In this study, the self-tuning intelligence digital twin is presented for bearing pattern recogn...
In this research, advanced technology is used to monitoring chaotic time-series signals. The combination of autoregressive with adaptive network-fuzzy algorithms is suggested for chaotic signal prediction. The autoregressive prediction algorithm is recommended for chaotic time-series prediction. This technique is linear, and the modeling prediction...
In this research, the combination of the smart digital twin (SDT) and the machine learning technique is prescribed to have a reliable fault pattern recognition in this effort. In the first stage, the SDT for the bearing is designed by the dynamical system modeling, updated using the data-driven autoregression approach, and estimate the performance...
This paper proposes an accurate and stable gearbox fault diagnosis scheme that combines a localized adaptive denoising technique with a wavelet-based vibration imaging approach and a deep convolution neural network model. Vibration signatures of a gearbox contain important fault-related information. However, this useful fault-related information is...
In this research, the aim is to investigate an adaptive digital twin algorithm for fault diagnosis and crack size identification in bearings. The main contribution of this research is to design an adaptive digital twin (ADT). The design of the ADT technique is based on two principles: normal signal modeling and estimation of signals. A combination...
Pipelines are a nonlinear and complex component to transfer fluid or gas from one place to another. From economic and environmental points of view, the safety of transmission lines is incredibly important. Furthermore, condition monitoring and effective data analysis are important to leak detection and localization in pipelines. Thus, an effective...
Pipelines are a nonlinear and complex component to transfer fluid or gas from one place to another. From economic and environmental points of view, the safety of transmission lines is incredibly important. Furthermore, condition monitoring and effective data analysis are important to leak detection and localization in pipelines. Thus, an effective...
Bearing is one of the most vital components of industrial machinery. The failure of bearing causes severe problems in the machinery. Therefore, continuous monitoring for the bearings is essential rather than regular manual checking, with the requirement for accuracy of prediction and efficiency. This paper proposes a novel intelligent bearing fault...
In this paper, an explainable AI-based fault diagnosis model for bearings is proposed with five stages, i.e., (1) a data preprocessing method based on the Stockwell Transformation Coefficient (STC) is proposed to analyze the vibration signals for variable speed and load conditions, (2) a statistical feature extraction method is introduced to captur...
Condition monitoring is used to track the unavoidable phases of rolling element bearings in an induction motor (IM) to ensure reliable operation in domestic and industrial machinery. The convolutional neural network (CNN) has been used as an effective tool to recognize and classify multiple rolling bearing faults in recent times. Due to the nonline...
In this study, the application of an intelligent digital twin integrated with machine learning for bearing anomaly detection and crack size identification will be observed. The intelligent digital twin has two main sections: signal approximation and intelligent signal estimation. The mathematical vibration bearing signal approximation is integrated...
Centrifugal pumps are important types of electro-mechanical machines used for fluid and energy conveyance. Mechanical faults in centrifugal pumps lead to abnormal impacts in the vibration signal of the system. Those impacts induce nonstationarity in vibration signals and hence complex time-frequency domain signal analysis techniques are required to...
Centrifugal pumps are the most vital part of any process industry. A fault in centrifugal pump can affect imperative industrial processes. To ensure reliable operation of the centrifugal pump, this paper proposes a novel automated health state diagnosis framework for centrifugal pump that combines a signal to time-frequency imaging technique and an...
Bearings are complex components with onlinear behavior that are used to mitigate the effects of inertia. These components are used in various systems, including motors. Data analysis and condition monitoring of the systems are important methods for bearing fault diagnosis. Therefore , a deep learning-based adaptive neural-fuzzy structure technique...
This paper introduces a novel approach to crack detection and localization in a pipeline transporting fluid under high pressure. From acoustic emission signals acquired by two R15i-AST sensors at two ends of a fluid pipeline, the proposed method scans peaks in the individual signal channels in the time-frequency domain and filters out noise to obta...
This paper proposes a reliable fault identification model of multi-level gearbox defects by applying adaptive noise control and a genetic algorithm-based feature selection for extracting the most related fault components of the gear vibration characteristic. The adaptive noise control analyzes the gearbox vibration signals to remove multiple noise...
Bearing failure generates burst signals when the rolling elements touch a crack on the outer race or inner race of the bearing. Over a decade, the acoustic emission (AE) has been used for detecting low-speed bearing failures. Since high sampling rate of the AE signal makes it difficult to design and extract good features, deep neural network-based...
This paper introduces a technique using a k-nearest neighbor (k-NN) classifier and hybrid features extracted from acoustic emission (AE) signals for detecting leakages in a gas pipeline. The whole algorithm is embedded in a microcontroller unit (MCU) to detect leaks in real-time. The embedded system receives signals continuously from a sensor mount...
. Rolling element bearing (REB) represent a class of nonlinear and multiple-degrees-of-freedom rotating machines that have pronounced coupling effects and can be used in various industries. The challenge of understanding complexity in a bearing’s dynamic behavior, coupling effects, and sources of uncertainty presents substantial challenges regardin...
Inner, outer, and ball faults are complex non-stationary and non-linear faults that occurs in rotating machinery such as bearings. Designing an effective procedure for fault diagnosis (FD) is essential to safe operation of bearings. To address fault diagnosis issue, a robust, hybrid technique based on the ARX-Laguerre fuzzy-sliding proportional int...
Bearings are complex components with nonlinear behavior that are used to reduce the effect of inertia. They are used in applications such as induction motors and rotating components. Condition monitoring and effective data analysis are important aspects of fault detection and classification in bearings. Thus, an effective and robust hybrid techniqu...
Inner, outer, and ball faults are complex non-stationary and non-linear faults that occurs in rotating machinery such as bearings. Designing an effective procedure for fault diagnosis (FD) is essential to safe operation of bearings. To address fault diagnosis issue, a robust, hybrid technique based on the ARX-Laguerre fuzzy-sliding proportional int...
Feature analysis plays an important role in determining the various health conditions of mechanical vessels. To achieve balance between traditional feature extraction and the automated feature selection process, a hybrid bag of features (HBoF) is designed for the health state classification of spherical tanks in this paper. The proposed HBoF is com...
Condition monitoring of induction motors plays a significant role in avoiding unexpected breakdowns and reducing excessive maintenance costs. In the majority of cases, bearing faults are found to be an issue in the failure of induction motors. The detection and valuation of irregularities at an early stage can help prevent disastrous failures. In t...
Bearings are complex components with nonlinear behavior that are used to reduce the effect of inertia. They are used in applications such as induction motors and rotating components. Condition monitoring and effective data analysis are important aspects of fault detection and classification in bearings. Thus, an effective and robust hybrid techniqu...
This paper establishes a methodology to exploit the characteristics of burst waveform in acoustic emission (AE) signals and combine with the signal analysis process to enhance the accuracy of multi-level leak detection in steel pipelines. The AE bursts are signal waveforms that contain the continual imbrication transients with variable strengths in...
Gearbox fault diagnosis based on the analysis of vibration signals has been a major research topic for a few decades due to the advantages of vibration characteristics. Such characteristics are used for early fault detection to guarantee the enhanced safety of complex systems and their cost-effective operation. There exist many fault diagnosis mode...
Rolling element bearings are a vital part of rotating machines and their sudden failure can result in huge economic losses as well as physical causalities. Popular bearing fault diagnosis techniques include statistical feature analysis of time, frequency, or time-frequency domain data. These engineered features are susceptible to variations under i...
This paper proposes a three-stage fault diagnosis strategy for multistage centrifugal pumps. First, the proposed method identifies and selects fault characteristic modes of vibration to overcome the substantial noise produced by other unrelated macro-structural vibrations. In the second stage, raw hybrid statistical features are extracted from the...
A new method is established to construct the 2-D fault diagnosis representation of multiple bearing defects from 1-D acoustic emission signals. This technique starts by applying envelope analysis to extract the envelope signal. A novel strategy is propounded for the deployment of the continuous wavelet transform with damage frequency band informati...