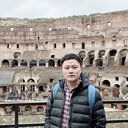
Haitao WangUniversity of Greifswald · Institute for Microbiology
Haitao Wang
Ph.D
About
56
Publications
49,748
Reads
How we measure 'reads'
A 'read' is counted each time someone views a publication summary (such as the title, abstract, and list of authors), clicks on a figure, or views or downloads the full-text. Learn more
879
Citations
Introduction
Additional affiliations
April 2018 - present
October 2015 - October 2017
September 2013 - December 2017
Education
September 2011 - December 2017
September 2007 - June 2011
Publications
Publications (56)
Urban greenspaces provide extensive ecosystem services, including pollutant remediation, water management, carbon maintenance, and nutrient cycling. However, while the urban soil microbiota underpin these services, we still have limited understanding of the factors that influence their distribution. We characterized soil bacterial communities from...
Coastal ecosystems are hotspots for nitrogen cycling, and specifically for nitrogen removal from water and sediment through the coupled nitrification-denitrification process. Salinity is globally important in structuring bacterial and archaeal communities, but the association between salinity and microbially-mediated nitrification and denitrificati...
Background
Paddy soil dissolved organic matter (DOM) represents a major hotspot for soil biogeochemistry, yet we know little about its chemodiversity let alone the microbial community that shapes it. Here, we leveraged ultrahigh-resolution mass spectrometry, amplicon, and metagenomic sequencing to characterize the molecular distribution of DOM and...
Transition of populations from rural to urban living causes landscape changes and alters the functionality of soil ecosystems. It is unclear how this urbanization disturbs the microbial ecology of soils and how the disruption influences nitrogen cycling. In this study, microbial communities in turfgrass-grown soils from urban and suburban areas aro...
The rewetting of formerly drained peatlands can help to counteract climate change through the reduction of CO 2 emissions. However, this can lead to resuming CH 4 emissions due to changes in the microbiome, favoring CH 4-producing archaea. How plants, hydrology and microbiomes interact as ultimate determinants of CH 4 dynamics is still poorly under...
Permafrost soils in the northern hemisphere are known to harbor large amounts of soil organic matter (SOM). Global climate warming endangers this stable soil organic carbon (SOC) pool by triggering permafrost thaw and deepening the active layer, while at the same time progressing soil formation. But depending, e.g., on ice content or drainage, cond...
Kelp deforestation by sea urchin grazing is a widespread phenomenon globally, with vast consequences for coastal ecosystems. The ability of sea urchins to survive on a kelp diet of poor nutritional quality is not well understood and bacterial communities in the sea urchin intestine may play an important role in digestion. A no-choice feeding experi...
Background
Methanogenic archaea represent a less investigated and likely underestimated part of the intestinal tract microbiome in swine.Aims/Methods
This study aims to elucidate the archaeome structure and function in the porcine intestinal tract of healthy and H1N1 infected swine. We performed multi-omics analysis consisting of 16S rRNA gene prof...
The hydrogen-dependent and methylotrophic order Methanomassiliicoccales consists of the families Methanomethylophilaceae and Methanomassiliicoccaceae. While Methanomethylophilaceae are comparatively well studied, there is a lack of knowledge on Methanomassiliicoccaceae. In this 16S rRNA gene amplicon sequencing based study we investigated the tempo...
The rewetting of formerly drained peatlands is a strategy to fight against global warming through the reduction of CO2 emissions, although this can lead to elevated CH4 emissions. The interplay between plants, hydrology and microbiomes as ultimate determinants of CH4 dynamics is still poorly understood, despite recent progress in field studies.
Usi...
The rewetting of formerly drained peatlands is a strategy to fight against global warming through the reduction of CO2 emissions, although this can lead to elevated CH4 emissions. The interplay between plants, hydrology and microbiomes as ultimate determinants of CH4 dynamics is still poorly understood, despite recent progress in field studies.
Usi...
Rewetted peatlands are reestablished hot spots for CH4 emissions, which are subject to increased drought events in the course of climate change. However, the dynamics of soil methane-cycling microbiomes in rewetted peatlands during summer drought are still poorly characterized. Using a quantitative metatranscriptomic approach, we investigated the c...
In temperate regions climate warming alters temperature and precipitation regimes. During winter, a decline in insulating snow cover changes the soil environment, where especially frost exposure can have severe implications for soil microorganisms and subsequently for soil nutrient dynamics. Here we investigated winter climate change responses in E...
Influenza A Virus (IAV) infection followed by bacterial pneumonia often leads to hospitalization and death in individuals from high risk groups. Following infection, IAV triggers the process of viral RNA replication which in turn disrupts healthy gut microbial community, while the gut microbiota plays an instrumental role in protecting the host by...
Different proxies for changes in structure and/or function of microbiomes have been developed, allowing assessing microbiome dynamics at multiple levels. However, the lack and differences in understanding the microbiome dynamics are due to the differences in the choice of proxies in different studies and the limitations of proxies themselves. Here,...
The full genome of a Methanomassiliicoccales strain, U3.2.1, was obtained from enrichment cultures of percolation fen peat soil under methanogenic conditions, with methanol and hydrogen as the electron acceptor and donor, respectively. Metagenomic assembly of combined long-read and short-read sequences resulted in a 1.51-Mbp circular genome.
Seasonal influenza outbreaks represent a large burden for the health care system as well as the economy. While the role of the microbiome has been elucidated in the context of various diseases, the impact of respiratory viral infections on the human microbiome is largely unknown. In this study, swine was used as an animal model to characterize the...
A highly resolved taxonomy for ammonia-oxidizing archaea (AOA) based on the alpha subunit of ammonia monooxygenase (amoA) was recently established, which uncovered novel environmental patterns of AOA, challenging previous generalizations. However, many microbiome studies target the 16S rRNA gene as a marker; thus, the usage of this novel taxonomy i...
In the last decades, rewetting of drained peatlands is on the rise worldwide, to restore their significant carbon sink function. Despite the increasing understanding of peat microbiomes, little is known about the seasonal dynamics and network interactions of the microbial communities in these ecosystems, especially in rewetted fens (groundwater-fed...
Swine are regarded as promising biomedical models, but the dynamics of their gastrointestinal microbiome have been much less investigated than that of humans or mice. The aim of this study was to establish an integrated multi-omics protocol to investigate the fecal microbiome of healthy swine. To this end, a preparation and analysis protocol includ...
An amendment to this paper has been published and can be accessed via the original article.
Aerated topsoils are important sinks for atmospheric methane (CH4) via oxidation by CH4‐oxidizing bacteria (MOB). However, intensified management of grasslands and forests may reduce the CH4 sink capacity of soils. We investigated the influence of grassland land‐use intensity (150 sites) and forest management type (149 sites) on potential atmospher...
Drained peatlands are significant sources of the greenhouse gas (GHG) carbon dioxide. Rewetting is a proven strategy used to protect carbon stocks; however, it can lead to increased emissions of the potent GHG methane. The response to rewetting of soil microbiomes as drivers of these processes is poorly understood, as are the biotic and abiotic fac...
Of all terrestrial ecosystems, peatlands store carbon most effectively in long-term scales of millennia. However, many peatlands have been drained for peat extraction or agricultural use. This converts peatlands from sinks to sources of carbon, causing approx. 5% of the anthropogenic Soil Syst. 2020, 4, 14 2 of 27 greenhouse effect and additional n...
In the last decades, rewetting of drained peatlands is on the rise worldwide, to restore the significant carbon sink function. Rewetted peatlands differ substantially from their pristine counterparts and can, thus, be considered as novel ecosystems. Despite the increasing understanding of peat microbiomes, little is known about the seasonal dynamic...
Drained peatlands are significant sources of the greenhouse gas (GHG) carbon dioxide. Rewetting is a proven strategy to protect carbon stocks; however, it can lead to increased emissions of the potent GHG methane. The response to rewetting of soil microbiomes as drivers of these processes is poorly understood, as are biotic and abiotic factors that...
Of all terrestrial ecosystems, peatlands store carbon most effectively. However, many peatlands have been drained for peat extraction or agricultural use. This converts peatlands from sinks to sources of carbon, causing approx. 5% of the anthropogenic greenhouse effect and additional negative effects on other ecosystem services. Rewetting peatlands...
High-organic drained peatlands are large sources of the greenhouse gas (GHG) carbon dioxide. Rewetting of peatlands is a promising strategy to protect the large C stocks, however, rewetting also causes increased emissions of the potent GHG methane. The net climate effect of rewetting and the role of the microbiome remains uncertain. We aimed at inv...
A recent study by Alves et al. (2018) established a highly resolved ammonia-oxidizing archaea (AOA) taxonomy based on the marker gene for the alpha subunit of ammonia monooxygenase (amoA) and uncovered novel environmental patterns of AOA that challenged previous generalizations. However, many microbiome studies target the 16S rRNA gene as marker, t...
Drained peatlands are significant sources of the greenhouse gas (GHG) carbon dioxide. Rewetting is a proven strategy to protect carbon stocks; however, it can lead to increased emissions of the potent GHG methane. The response to rewetting of soil microbiomes as drivers of these processes is poorly understood, as are biotic and abiotic factors that...
The consequences of urbanisation for Earth's biogeochemical cycles are largely unexplored. Copper (Cu) in urban soils is being accumulated mainly due to anthropogenic activities under rapid urbanisation. The increasing Cu concentrations may contribute to altering soil nitrogen (N) cycling in urban ecosystems through modulating denitrification proce...
High-organic drained peatlands are large sources of the greenhouse gas (GHG) carbon dioxide. Rewetting of peatlands is a promising strategy to protect the large C stocks, however, rewetting also causes increased emissions of the potent GHG methane. The net climate effect of rewetting and the role of the microbiome remains uncertain. We aimed at inv...
Peatlands as the major soil carbon reservoirs are widely distributed in Northern Germany. Recent drainage of high-organic peat-soils for agricultural purposes has led to increased greenhouse gas emissions and loss of biodiversity. Rewetting of drained fens and paludiculture (“wetland farming”) are introduced to mitigate these negative impacts. Howe...
Tidal mangrove wetlands are a source of methane (CH4) and nitrous oxide (N2O); but considering the high productivity of mangroves, they represent a significant sink for carbon dioxide (CO2). An exotic plant Spartina alterniflora has invaded east China over the last few decades, threatening these coastal mangrove ecosystems. However, the atmospheric...
Purpose The aim of this study was to elucidate the impact of plant on the activity, abundance, and the community composition of the ammonia oxidizers, including both ammonia-oxidizing archaea (AOA) and bacteria (AOB). Moreover, the relationship between AOA and AOB in mangrove sediment was also analyzed. Materials and methods Sediment used for micro...
Coastal mangrove wetland is well known to be intense in nitrogen cycling. The tidal process and plants are key factors in controlling the microbial processes in wetlands. However, mechanisms on how these factors and their interactions affect the microorganisms involved in denitrification remain poorly understood. In this study, the impacts of veget...
Phaeocystis globosa blooms have frequently occurred along coastal waters and exerted serious impacts on ecological environments by releasing toxic hemolytic substances, forming nuisance foam, and causing oxygen depletion. An actinomycete strain RPS with high algicidal activity against P. globosa was isolated and identified as Streptomyces alboflavu...
To evaluate the effects of environmental factors on the greenhouse gases (GHGs) fluxes, sediment samples from the Mangrove Nature Reserve of Jiulong River estuary were collected and homogenized evenly before microcosm experiments. Tide and vegetation types were simulated in the microcosm. A static chamber technique was used to measure the fluxes of...
Denitrification, a microbial redox process in which nitrogen oxides are reduced stepwise to gaseous products, is an important step in nitrogen cycling, especially in the soil environments. Denitrifying microbes possess a series of enzymes encoded by various functional denitrifying genes. The classic 16S rRNA molecular method is not suitable for the...
Questions
Questions (9)
We recently got our sequencing data from a company sequenced with Novaseq. As far as I have learnt, Novaseq uses a simpler quanlity score system, which merges different scores into only four categories/levels. This of course causes problem when processing the data with quality-score based pipeline, e.g. DADA2. I got really weired results with DADA2. We also tried with UNOISE3, the ASV table looks fine for the mock data. But since this is also based on quanlity, I'm not sure if this can be trusted.
I also searched for some publications using Novaseq for amplicon sequencing. They all used traditional/old pipelines based on similarity to generate OTUs. I wonder if this is the only way to do this.
I wish there could be someone who has experience dealing with this situation. Is there still some possible way to use DADA2 or UNOISE3 with some modifications to process the data?
I have a dataset, assoicated with 5 time points. For each time point, I have three replicates. I understand that for a time-series dataset, repeated-measures ANOVA or Friedman test would be the best solution.
However, the three replicates are totally random. Let's say, I measured the gas flux from three random locations in a small region (20*20 square meters) at each time point. Even though the measurement was done in the same region, I can't relate these random replicates as three sets.
What I did was using independent ANOVA and using time points as the groups. But I know this is inappropriate. Anyone knows if there is an appropriate method for dealing with this situation?
I have hundreds of samples with different sample sizes/counts (3,000 to 150,000). Due to the uneven sizes, comparing the richness between samples can be tricky without rarefying. However, if I rarefy the data to 3,000 counts per sample, many samples' richness would drop significantly, which is also tricky. I wonder if I should use the rarefied richness or the normal richness for data interpretation.
I personally don't like rarefying, which might throw away many rare taxa. I know Shannon which takes into account the relative abundance of each species can to some extent solve this problem. But what if I'm specifically interested in the richness?
I hope someone could provide some enlightening comments on this.
I have created co-occurrence network based on Spearman's correlations for several times with different projects. My research focuses on microbiome. So the networks were the correlations between different microbial taxa. When I filtered the correlations by coefficients above 0.7 or 0.8, I only got positive correlations (or at least most of them positive correlations). My colleagues also got the same issue as me. After I checked many literatures, they also got like mostly the positive correlations based on filtering with a high cutoff of coefficients.
Is there any reason why this happened? Then how to interpret from all positive correlations?
I'm quite confused about using DESeq2 to find the differential abundant taxa in microbiome studies, especially when there are more than two groups of the factor. I know DESeq2 was initially used for RNA-seq to detect the regulation of gene expressions. It's easy to understand when there are only two groups, e.g. treated vs. untreated. We can easily say which taxa was up-regulated by looking at the log2fold change (positive or negative).
BUT what about when there are three groups, control vs. treat1 vs. treat2? The DESeq2 can still handle this situation, but then I have no idea how to interpret the log2fold change. If we detected some taxa that were significantly different, how can we know in which group these taxa were up-regulated?
Although some people suggest to do the pairwise comparison, it's still unclear to me how to do it and how to interpret it. Does anyone have very good recommendation or idea about this?
Thanks in advance.
I always have some problems with the formula while doing PERMANOVA. For example, adonis(distance.bray ~ x+y, sample.data, permutations = 999) and adonis(distance.bray ~ y+x, sample.data, permutations = 999) gave me the different results of the R square values for factor x and y. So what are the difference between these two formula? And which one should I trust?
Also, I once did the analysis with three factors. When I did adonis(distance.bray ~ x+y+z, sample.data, permutations = 999), z was significant. But when I do adonis(distance.bray ~ z, sample.data, permutations = 999), z was not significant. Can someone explain what happened in these analyses?
I'm wondering if there is a clear method that can be used to create the bipartite network to connect the samples with OTUs/taxa. As I searched the internet, all of the tutorials are talking about how to plot it but not how to calculate or how to get the associations between samples and taxa. Also, the papers that used bipartite network are unclear about the methods either. Although the qiime have the function to get the nodes and edges for bipartite (http://qiime.org/scripts/make_bipartite_network.html), it is unclear how qiime does the analysis. So what is the method/algorithm behind this?
I used adonis to test the difference between groups/categories based on the 16s high-throughput sequencing data. I got a very significant result (p = 0.001), but the R2 is very low (< 0.1). I know this means this model only explains less than 10 percent of my data. From the PCoA plot, there were some overlaps between the groups. Do these overlaps contribute to the low R2? I'm wondering if I can still say that the difference between groups is significant?
I also did ANOSIM and the results are similar. The statistic value is very low (~0.1) but p-value is 0.001. Is this the same problem I got when doing adonis?
Some discussion groups said that there is no relationship between R2 and p-value. Doesn't it matter however small the R2 is?