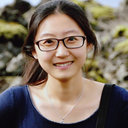
Fei ZhuTianjin University | tju · Center for Applied Mathematics
Fei Zhu
Doctor of Philosophy
About
20
Publications
3,474
Reads
How we measure 'reads'
A 'read' is counted each time someone views a publication summary (such as the title, abstract, and list of authors), clicks on a figure, or views or downloads the full-text. Learn more
294
Citations
Introduction
Additional affiliations
November 2016 - present
October 2013 - September 2016
Publications
Publications (20)
DNA has immense potential as an emerging data storage medium. The principle of DNA storage is the conversion and flow of digital information between binary code stream, quaternary base, and actual DNA fragments. This process will inevitably introduce errors, posing challenges to accurate data recovery. Sequence reconstruction consists of inferring...
Recent advances in neural networks have made great progress in the hyperspectral image (HSI) classification. However, the overfitting effect, which is mainly caused by complicated model structure and small training set, remains a major concern. Reducing the complexity of the neural networks could prevent overfitting to some extent, but also decline...
More recently, the multilinear mixing model (MLM) has been proposed in [4], and become a new research hotspot for nonlinear unmixing [5], [6]. The MLM accounts for all degrees of interactions between endmembers, and an additional parameter is introduced at each pixel to characterize the probability of further interactions. As this paper aims to imp...
Recent years has witnessed the success of convolutional neural networks (CNNs) in many machine learning and pattern recognition applications, especially in image recognition. However, due to the increasing model complexity, the parameter redundancy problem arises, and greatly degrades the performance of CNNs. To alleviate this problem, various regu...
Recently, many methods based on deep learning (DL) have been used for hyperspectral image (HSI) classification and achieved good performance. But such approaches often need numerous labelled training samples. This issue is aggravated when applying DL on small-scale HSIs. To alleviate this problem, transfer learning (TL) is introduced to HSI analysi...
Recent advances in neural networks have made great progress in the hyperspectral image (HSI) classification. However, the overfitting effect, which is mainly caused by complicated model structure and small training set, remains a major concern. Reducing the complexity of the neural networks could prevent overfitting to some extent, but also decline...
To improve the classification performance in the context of hyperspectral image (HSI) processing, many works have been developed based on two common strategies, namely, the spatial-spectral information integration and the utilization of neural networks. However, both strategies typically require more training data than the classical algorithms, agg...
Spectral unmixing of hyperspectral images is an important issue in the fields of remote sensing. Jointly exploring the spectral and spatial information embedded in the data is helpful to enhance the consistency between mixing/unmixing models and real scenarios. This paper proposes a graph regularized nonlinear unmixing method based on the recent mu...
To improve the classification performance in the context of hyperspectral image processing, many works have been developed based on two common strategies, namely the spatial-spectral information integration and the utilization of neural networks. However, both strategies typically require more training data than the classical algorithms, aggregatin...
The shortage of training samples remains one of the main obstacles in applying the artificial neural networks (ANN) to the hyperspectral images classification. To fuse the spatial and spectral information, pixel patches are often utilized to train a model, which may further aggregate this problem. In the existing works, an ANN model supervised by c...
In this paper, we propose a spectral-spatial feature extraction and classification framework based on artificial neuron network (ANN) in the context of hyperspectral imagery. With limited labeled samples, only spectral information is exploited for training and spatial context is integrated posteriorly at the testing stage. Taking advantage of recen...
Nonnegative matrix factorization (NMF) has become a prominent signal processing and data analysis technique. To address streaming data, online methods for NMF have been introduced recently, mainly restricted to the linear model. In this paper, we propose a framework for online nonlinear NMF, where the factorization is conducted in a kernel-induced...
In hyperspectral images, some spectral bands suffer from low signal-to-noise ratio due to noisy acquisition and atmospheric effects, thus requiring robust techniques for the unmixing problem. This paper presents a robust supervised spectral unmixing approach for hyperspectral images. The robustness is achieved by writing the unmixing problem as the...
Nonnegative matrix factorization (NMF) has been increasingly investigated for data analysis and dimension-reduction. To tackle large-scale data, several online techniques for NMF have been introduced recently. So far, the online NMF has been limited to the linear model. This paper develops an online version of the nonlinear kernel-based NMF, where...
Nonnegative matrix factorization (NMF) is a powerful class of feature
extraction techniques that has been successfully applied in many fields, namely
in signal and image processing. Current NMF techniques have been limited to a
single-objective problem in either its linear or nonlinear kernel-based
formulation. In this paper, we propose to revisit...
The nonnegative matrix factorization (NMF) is widely used in signal and image processing, including bio-informatics, blind source separation and hyperspectral image analysis in remote sensing. A great challenge arises when dealing with nonlinear NMF. In this paper, we propose an efficient nonlinear NMF, which is based on kernel machines. As opposed...
The nonnegative matrix factorization (NMF) is widely used in signal and image
processing, including bio-informatics, blind source separation and
hyperspectral image analysis in remote sensing. A great challenge arises when
dealing with a nonlinear formulation of the NMF. Within the framework of kernel
machines, the models suggested in the literatur...