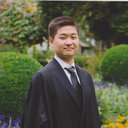
Dongda ZhangThe University of Manchester · School of Chemical Engineering and Analytical Science
Dongda Zhang
PhD, University of Cambridge
About
105
Publications
21,576
Reads
How we measure 'reads'
A 'read' is counted each time someone views a publication summary (such as the title, abstract, and list of authors), clicks on a figure, or views or downloads the full-text. Learn more
1,987
Citations
Introduction
Process Modelling, Optimisation, and Design for Sustainable Polymers Production
Additional affiliations
February 2018 - present
Position
- Fellow
Description
- I am an Honorary Research Fellow at the Centre for Process Systems Engineering, collaborating closely with other research fellows to develop advanced technologies for bioprocess optimisation.
October 2016 - January 2018
Position
- PostDoc Position
Description
- My project works on the development of process modelling, design and optimisation methods for exploring the economics, scalability and sustainability of new routes to polymers which combine bio-based feedstocks and carbon dioxide.
Education
October 2013 - June 2016
October 2012 - September 2013

Independent Researcher
Field of study
- Chemical Engineering
Publications
Publications (105)
The industrialization of catalytic processes requires reliable kinetic models for their design, optimization and control. Mechanistic models require significant domain knowledge, while data-driven and hybrid models lack interpretability. Automated knowledge discovery methods, such as ALAMO (Automated Learning of Algebraic Models for Optimization),...
Machine learning models for chemical retrosynthesis have attracted substantial interest in recent years. Unaddressed challenges, particularly the absence of robust evaluation metrics for performance comparison, and the lack of black-box interpretability, obscure model limitations and impede progress in the field. We present an automated benchmarkin...
Machine learning models for chemical retrosynthesis have attracted substantial interest in recent years. Unaddressed challenges, particularly the absence of robust evaluation metrics for performance comparison, and the lack of black-box interpretability, obscure model limitations and impede progress in the field. We present an automated benchmarkin...
Machine learning models for chemical retrosynthesis have attracted substantial interest in recent years. Unaddressed challenges, particularly the absence of robust evaluation metrics for performance comparison, and the lack of black-box interpretability, obscure model limitations and impede progress in the field. We present an automated benchmarkin...
Over the last decade, there has been a significant shift from traditional mechanistic and empirical modelling into statistical and data-driven modelling for applications in reaction engineering. In particular, the integration of machine learning and first-principle models has demonstrated significant potential and success in the discovery of (bio)c...
Over the last decade, there has been a significant shift from traditional mechanistic and empirical modelling into statistical and data-driven modelling for applications in reaction engineering. In particular, the integration of machine learning and first-principle models has demonstrated significant potential and success in the discovery of (bio)c...
Over the last decade, there has been a significant shift from traditional mechanistic and empirical modelling into statistical and data-driven modelling for applications in reaction engineering. In particular, the integration of machine learning and first-principle models has demonstrated significant potential and success in the discovery of (bio)c...
Over the last decade, there has been a significant shift from traditional mechanistic and empirical modelling into statistical and data-driven modelling for applications in reaction engineering. In particular, the integration of machine learning and first-principle models has demonstrated significant potential and success in the discovery of (bio)c...
Biotechnological exploitation of fast-growing cyanobacterial species is hindered by unavailable mechanistic interpretations for the differing bioconversion rates when exploring strains with similar metabolic pathways and transport systems. This study investigated two strains: Synechococcus sp. PCC 11901, the fastest growing cyanobacterium identifie...
Optimisation and upscaling of biohydrogen production in externally pumped-recirculated photobioreactors (PBRs) are hampered by the infeasible computational cost of coupling hydrodynamics, to light transmission and bioreaction transport, through the column and peristaltic pump. This study approximates the complexities of peristaltic pump with inlet-...
The industrialization of catalytic processes is of far more importance today than it has ever been before and kinetic models are essential tools for their industrialization. Kinetic models affect the design, the optimization and the control of catalytic processes, but they are not easy to obtain. Classical paradigms, such as mechanistic modeling re...
Hybrid modelling combines data-driven and mechanistic modelling, providing a cost-effective solution to modelling complex biochemical reaction kinetics when the underlying mechanisms are not fully understood. However, the question of how much kinetic information to incorporate into a hybrid bioprocess model has not been systematically addressed. Th...
Constructing predictive models to simulate complex bioprocess dynamics, particularly time‐varying (i.e., parameters varying over time) and history‐dependent (i.e., current kinetics dependent on historical culture conditions) behavior, has been a longstanding research challenge. Current advances in hybrid modeling offer a solution to this by integra...
Viscosity represents a key product quality indicator but has been difficult to measure in-process in real-time. This is particularly true if the process involves complex mixing phenomena operated at dynamic conditions. To address this challenge, in this study, we developed an innovative soft sensor by integrating advanced artificial neural networks...
The major promise of the 4th industrial revolution is encompassed by the utilization of real-time process data to inform operational decision-making. Research focus relating to the monitoring of batch process end product qualities has typically been dominated by the use of latent variable models. In this work, we combined latent variable modeling w...
Chemical process optimization and control are affected by (1) plant-model mismatch, (2) process disturbances, and (3) constraints for safe operation. Reinforcement learning by policy optimization would be a natural way to solve this due to its ability to address stochasticity, plant-model mismatch, and directly account for the effect of future unce...
Reinforcement Learning (RL) has recently received significant attention from the process systems engineering and control communities. Recent works have investigated the application of RL to identify optimal scheduling decision in the presence of uncertainty. In this work, we present a RL methodology to address precedence and disjunctive constraints...
Dynamic flux analysis methods have been widely used for deciphering complex metabolic fluxes transients. However, many of them require frequent experimental measurements and are ineffective in dealing with under-determined metabolic reaction networks. In this study, we addressed these challenges by (i) integrating a macroscale kinetic model with it...
In the literature, machine learning (ML) and artificial intelligence (AI) applications tend to start with examples that are irrelevant to process engineers (e.g. classification of images between cats and dogs, house pricing, types of flowers, etc.). However, process engineering principles are also based on pseudo-empirical correlations and heuristi...
The field of Reinforcement Learning (RL) has received a lot of attention for decision-making under uncertainty. Lately, much of this focus has been on the application of RL for combinatorial optimisation. Recent work has showcased the use of RL on a single-stage continuous chemical production scheduling problem. This work highlighted the potential...
Reinforcement Learning (RL) has generated excitement within the process industries within the context of decision making under uncertainty. The primary benefit of RL is that it provides a flexible and general approach to handling systems subject to both exogenous and endogenous uncertainties. Despite this there has been little reported uptake of RL...
Statistical machine learning algorithms have been widely used to analyse industrial data for batch process monitoring and control. In this study, we develop a three-step methodology to identify, visualize and systematically reduce data dimensionality for the construction of robust soft-sensors for end-product quality prediction. The approach first...
Reinforcement Learning (RL) has received interest within the context of decision making under uncertainty in the process industries. The primary benefit of RL arises from the formulation of the control problem as a Markov decision process (MDP), meaning that it inherits the benefits of accounting for uncertainty in a closed loop feedback control fr...
Viscosity represents a key indicator of product quality but has traditionally been difficult to measure in-process in real-time. This is particularly true if the process involves complex mixing phenomena operated at dynamic conditions. To address this challenge, a promising solution to monitoring product viscosity is to design soft-sensors which co...
Microbiological production of γ-linolenic acid (GLA) via a temperature-shift strategy has been found to shorten the batch cultivation period, improve GLA yields and reduce the operational cost. However, the underlining biochemical mechanistic phenomena are highly complex and challenging to model, thus hindering commercial upscaling applications of...
Background: Parallel to the impending hydrogen economy, the simultaneous treatment of organic waste and biohydrogen synthesis by the photosynthetic bacterium Rhodopseudomonaspalustris is a promising renewable energy technology. However, studies so far have been mostly laboratory based with upscaling of the bioprocess still being an open challenge....
Reinforcement learning (RL) is a control approach that can handle nonlinear stochastic optimal control problems. However, despite the promise exhibited, RL has yet to see marked translation to industrial practice primarily due to its inability to satisfy state constraints. In this work we aim to address this challenge. We propose an “oracle”-assist...
Predictive modeling of new biochemical systems with small data is a great challenge. To fill this gap, transfer learning, a subdomain of machine learning that serves to transfer knowledge from a generalized model to a more domain‐specific model, provides a promising solution. While transfer learning has been used in natural language processing, ima...
Astaxanthin is a high‐value compound commercially synthesized through Xanthophyllomyces dendrorhous fermentation. Using mixed sugars decomposed from biowastes for yeast fermentation provides a promising option to improve process sustainability. However, little effort has been made to investigate the effects of multiple sugars on X. dendrorhous biom...
Statistical machine learning algorithms have been widely used to analyse industrial data for batch process monitoring and control. In this study, we aimed to take a two-step approach to systematically reduce data dimensionality and to design soft-sensors for product quality prediction. The approach first employs partial least squares to screen the...
Developing kinetic models to simulate Rhodopseudomonas palustris biohydrogen production within different configurations of photobioreactors (PBRs) poses a significant challenge. In this study, two types of PBRs: schott bottle-based and vertical tubular-based, were investigated, and three original contributions are presented. Firstly, a mechanistic...
Most optimization problems in engineering can be formulated as ‘expensive’ black box problems whose solutions are limited by the number of function evaluations. Frequently, engineers develop accurate models of physical systems that are differentiable and/or cheap to evaluate. These models can be solved efficiently, and the solution transferred to t...
With liquefied natural gas becoming increasingly prevalent as a flexible source of energy, the design and optimization of industrial refrigeration cycles becomes even more important. In this article, we propose an integrated surrogate modeling and optimization framework to model and optimize the complex CryoMan Cascade refrigeration cycle. Dimensio...
Reinforcement learning (RL) is a data‐driven approach to synthesizing an optimal control policy. A barrier to wide implementation of RL‐based controllers is its data‐hungry nature during online training and its inability to extract useful information from human operator and historical process operation data. Here, we present a two‐step framework to...
The field of machine learning is comprised of techniques, which have proven powerful approaches to knowledge discovery and construction of ‘digital twins’ in the highly dimensional, nonlinear and stochastic domains common to biochemical engineering. We review the use of machine learning within biochemical engineering within the last 20 years. The m...
Reinforcement Learning (RL) controllers have generated excitement within the control community. The primary advantage of RL controllers relative to existing methods is their ability to optimize uncertain systems independently of explicit assumption of process uncertainty. Recent focus on engineering applications has been directed towards the develo...
Reinforcement Learning (RL) controllers have generated excitement within the control community. The primary advantage of RL controllers relative to existing methods is their ability to optimize uncertain systems independently of explicit assumption of process uncertainty. Recent focus on engineering applications has been directed towards the develo...
Light attenuation is a primary challenge limiting the upscaling of photobioreactors for sustainable bio‐production. One key to this challenge, is to model and optimise the light/dark cycles so that cells within the dark region can be frequently transferred to the light region for photosynthesis. Therefore, this study proposes the first mechanistic...
The development of advanced process control schemes is continuing driver of research within process systems engineering. In this work, we propose a framework, which leverages existing process data to automatically learn and update a control policy. This framework is underpinned by machine learning methods, namely, apprenticeship (AL) and reinforcem...
Chemical process optimization and control often require satisfaction of constraints for safe operation. Reinforcement learning (RL) has been shown to be a powerful control technique that can handle nonlinear stochastic optimal control problems. Despite this promise, RL has yet to see significant translation to industrial practice due to its inabili...
Model-free reinforcement learning has been recently investigated for use in chemical process control. Through the iterative creation of an approximate process model, control actions are able to be explored and optimal policies generated. Typically, this approximate process model has taken the form of a neural network that is continuously updated. H...
Reinforcement learning (RL) is a control approach that can handle nonlinear stochastic optimal control problems. However, despite the promise exhibited, RL has yet to see marked translation to industrial practice primarily due to its inability to satisfy state constraints. In this work we aim to address this challenge. We propose an 'oracle'-assist...
Nonlinear model predictive control (NMPC) is one of the few control methods that can handle multivariable nonlinear control systems with constraints. Gaussian processes (GPs) present a powerful tool to identify the required plant model and quantify the residual uncertainty of the plant-model mismatch. It is crucial to consider this uncertainty, sin...
Chemical process optimization and control are affected by 1) plant-model mismatch, 2) process disturbances, and 3) constraints for safe operation. Reinforcement learning by policy optimization would be a natural way to solve this due to its ability to address stochasticity, plant-model mismatch, and directly account for the effect of future uncerta...
Integrating physical knowledge and machine learning is a critical aspect of developing industrially focused digital twins for monitoring, optimisation, and design of microalgal and cyanobacterial photo‐production processes. However, identifying the correct model structure to quantify the complex biological mechanism poses a severe challenge for the...
In this paper, surrogate modelling and optimization is investigated for use in large scale chemical processes. A novel CryoMan cascade liquefied natural gas (LNG) refrigeration cycle is selected as the case study which has been highlighted for potential use within industry. Given its high nonlinearity and dimensionality (31 input variables and 20 o...
Dynamic real-time optimization (DRTO) is a challenging task due to the fact that optimal operating conditions must be computed in real time. The main bottleneck in the industrial application of DRTO is the presence of uncertainty. Many stochastic systems present the following obstacles: 1) plant-model mismatch, 2) process disturbances, 3) risks in...
Biomass derived polymer production processes have been extensively studied to substitute petrochemical based polymer synthesis routes. However, previous research predominantly focused on the synthesis of nitrogen-free polymers. Therefore, a novel superstructure reaction network was constructed in this study to convert different types of biomass was...
Current advances in computer-aided chemical process design and synthesis take advantage of surrogate modelling and superstructure optimization techniques. Conventionally, this is completed by using first-principle physical models or data-driven models to replace the original rigorous models for optimization and selection of a specific unit operatio...
In this paper, surrogate modelling and optimization is investigated for use in large scale chemical processes. A novel CryoMan cascade liquefied natural gas (LNG) refrigeration cycle is selected as the case study which has been highlighted for potential use within industry. Given its high nonlinearity and dimensionality (31 input variables and 20 o...
Dynamic real-time optimization (DRTO) is a challenging task due to the fact that optimal operating conditions must be computed in real time. The main bottleneck in the industrial application of DRTO is the presence of uncertainty. Many stochastic systems present the following obstacles: 1) plant-model mismatch, 2) process disturbances, 3) risks in...
Bioprocesses have received a lot of attention to produce clean and sustainable alternatives to fossil-based materials. However, they are generally difficult to optimize due to their unsteady-state operation modes and stochastic behaviours. Furthermore, biological systems are highly complex, therefore plant-model mismatch is often present. To addres...
Nonlinear model predictive control (NMPC) is one of the few control methods that can handle multivariable nonlinear control systems with constraints. Gaussian processes (GPs) present a powerful tool to identify the required plant model and quantify the residual uncertainty of the plant-model mismatch given its probabilistic nature . It is crucial t...
Nonlinear model predictive control (NMPC) is one of the few control methods that can handle multivariable nonlinear control systems with constraints. Gaussian processes (GPs) present a powerful tool to identify the required plant model and quantify the residual uncertainty of the plant-model mismatch given its probabilistic nature . It is crucial t...
The development of digital bioprocessing technologies is critical to operate modern industrial bioprocesses. This study conducted the first investigation on the efficiency of using physics‐based and data‐driven models for the dynamic optimisation of long‐term bioprocess. More specifically, this study exploits a predictive kinetic model and a cuttin...
Bioprocesses have received great attention from the scientific community as an alternative to fossil-based products by microorganisms-synthesised counterparts. However, bioprocesses are generally operated at unsteady-state conditions and are stochastic from a macro-scale perspective, making their optimisation a challenging task. Furthermore, as bio...
Model‐based online optimization has not been widely applied to bioprocesses due to the challenges of modeling complex biological behaviors, low‐quality industrial measurements, and lack of visualization techniques for ongoing processes. This study proposes an innovative hybrid modeling framework which takes advantages of both physics‐based and data...
Microalgal biofuels have not yet achieved wide‐spread commercialization, partially as a result of the complexities involved with designing and scaling up of their biosystems. The sparger design of a pilot‐scale photobioreactor (120 L) was optimized to enable the scale‐up of biofuel production. An integrated model coupling computational fluid dynami...
Bioprocesses have received a lot of attention to produce clean and sustainable alternatives to fossil-based materials. However, they are generally difficult to optimize due to their unsteady-state operation modes and stochastic behaviours. Furthermore, biological systems are highly complex, therefore plant-model mismatch is often present. To addres...
Identifying optimal photobioreactor configurations and process operating conditions is critical to industrialize microalgae‐derived biorenewables. Traditionally, this was addressed by testing numerous design scenarios from integrated physical models coupling computational fluid dynamics and kinetic modeling. However, this approach presents computat...
Microorganism production and remediation processes are of critical importance to the next generation of sustainable industries. Undertaking mathematical treatment of dynamic biosystems operating at any spatial or temporal scale is essential to guarantee their performance and safety. However, constructing physical models remains a challenge due to t...
Dynamic modeling is an important tool to gain better understanding of complex bioprocesses and to determine optimal operating conditions for process control. Currently, two modeling methodologies have been applied to biosystems: kinetic modeling, which necessitates deep mechanistic knowledge, and artificial neural networks (ANN), which in most case...
Currently, algal fuel research has commenced to shift towards genetically engineered mutants, able to express and excrete desired products directly into the culture. In this study, a mutant strain of Chlamydomonas reinhardtii, engineered for bisabolene (alternative biodiesel) excretion, was cultivated at different illumination and temperatures to i...
Sustainable polymers derived from biomass have been considered as promising candidates to reduce the dependency on fossil based polymers. In this study, a conceptual process design was conducted for citrus waste derived biopolymer production with energy co-generation, and its eco-friendliness was evaluated through life cycle assessment by compariso...
Biomass is a promising feedstock for the production of sustainable biopolymers, which could offer a significant reduction of the adverse environmental impacts associated with conventional petroleum-based polymers. To further evaluate their potential, this study investigated the environmental impacts associated with the production of the newly propo...
Photosynthetic bacterium Rhodobacter sphaeroides S10 was grown photoheterotrophically to produce hydrogen in three types of culture vessels having very different surface-to-volume ratios (S). The aim was to investigate the effect of the culture system geometry (low-aspect ratio cylindrical geometry, a rectangular or flat bottle geometry, and a tubu...
Achieving optimal nutrient concentrations is essential to increasing the biomass productivity of algal raceway ponds. Nutrient mixing or distribution in raceway ponds is significantly affected by hydrodynamic and geometric properties. The nutrient mixing in algal raceway ponds under the influence of hydrodynamic and geometric properties of ponds is...
C-phycocyanin is a high-value bioproduct synthesized by cyanobacterium Arthrospira platensis with a significant global market demand given its applications in the pharmaceutical, food and colorant industries. Unfortunately, its biosynthesis is currently characterized by low productivity and large uncertainty during the production process. High vari...
Biomass derived polymers are considered as promising candidates to replace petroleum based polymers due to their potential environmental friendliness. To facilitate their application, in this study, a newly proposed biopolymer, polylimonene carbonate, was chosen as the representative to investigate the environmental impacts of the biopolymer produc...
High variability and unreliable expectations on product yields substantially hinder the industrialization of microorganism derived biochemicals as they present a risk to the profitability and safety of the underlying systems. Therefore, in this work, we propose an optimization approach to determine the lower and upper product yield expectations for...
Biodiesel produced from microalgae has been extensively studied due to its potentially outstanding advantages over traditional transportation fuels. In order to facilitate its industrialisation and improve the process profitability, it is vital to construct highly accurate models capable of predicting the complex behaviour of the investigated biosy...
L-tryptophan is an essential amino acid widely used in food and pharmaceutical industries. However, its production via Escherichia coli fermentation suffers severely from both low glucose conversion efficiency and acetic acid inhibition, and to date effective process control methods have rarely been explored to facilitate its industrial scale produ...
Lutein is a high-value bioproduct synthesised by microalga Desmodesmus sp.. It has great potential for the food, cosmetics, and pharmaceutical industries. However, in order to enhance its productivity and to fulfil its ever-increasing global market demand, it is vital to construct accurate models capable of simulating the entire behaviour of the co...
Sustainable polymers derived from biomass have great potential to replace petrochemical based polymers and fulfill the ever-increasing market demand. To facilitate their industrialization, in this research, a comprehensive superstructure reaction network comprising a large number of reaction pathways from biomass to both commercialized and newly pr...
Sustainable polymers derived from biomass have been extensively investigated to replace petroleum-based polymers and fulfil the ever-increasing market demand. Because of the diversity of biomass and polymer categories, there exist a large number of synthesis routes from biomass to polymers. However, their productive and economic potential have neve...
Lutein is a high-value bioproduct synthesised by microalga Desmodesmus sp. In the current study two aspects of this process are thoroughly investigated: identifying the complex effects of light intensity and nitrate concentration on biomass growth and lutein synthesis, and constructing an accurate kinetic model capable of simulating the entire biop...
Hydrogen produced by microorganisms has been considered as a potential solution for sustainable hydrogen production for the future. In the current study, an advanced real-time optimisation methodology is developed to maximise the productivity of a 21-day fed-batch cyanobacterial hydrogen production process, which to the best of our knowledge has no...
C-Phycocyanin is a high-value bioproduct synthesized from cyanobacterium Arthrospira platensis. To facilitate its application, advanced dynamic models were built to simulate the complex effects of light intensity, light attenuation, and nitrate concentration on cell growth and pigment production. When these models were compared to the experimental...
This research explores the photofermentation of glycerol to hydrogen by Rhodopseudomonas palustris, with the objective to maximise hydrogen production. Two piecewise models are designed to simulate the entire growth phase of R. palustris; a challenge that few dynamic models can accomplish. The parameters in both models were fitted by the present ba...
This paper presents a novel structured dynamic model to simulate the metabolic reaction network of green algae hydrogen production from aerobic cultivation conditions to anaerobic cultivation conditions, which has not been addressed in the open literature to this date. An efficient parameter estimation methodology is proposed to avoid the difficult...
This paper investigates the scaling-up of cyanobacterial biomass cultivation and biohydrogen production from laboratory to industrial scale. Two main aspects are investigated and presented, which to the best of our knowledge have never been addressed, namely the construction of an accurate dynamic model to simulate cyanobacterial photo-heterotrophi...
Hydrogen produced by microalgae is intensively researched as a potential alternative to conventional energy sources. Scaling-up of the process is still an open issue, and to this end accurate dynamic modeling is very important. A challenge in the development of these highly nonlinear dynamic models is the estimation of the associated kinetic parame...
Dynamic simulation is a valuable tool to assist the scale-up and transition of biofuel production from laboratory scale to potential industrial implementation. In the present study two dynamic models are constructed, based on the Aiba equation, the improved Lambert–Beer's law and the Arrhenius equation. The aims are to simulate the effects of incid...
Chlamydomonas reinhardtii is a green microalga with the potential to generate sustainable biofuels for the future. Process simulation models are required to predict the impact of laboratory-scale growth experiments on future scaled-up system operation. Two dynamic models were constructed to simulate C. reinhardtii photo-autotrophic and photo-mixotr...
Cyanothece sp. ATCC 51142 is considered a microorganism with the potential to generate sustainable hydrogen in the future. However, few kinetic models are capable of simulating different phases of Cyanothece sp. ATCC 51142 from growth to hydrogen production. In the present study four models are constructed to simulate Cyanothece sp. batch photoprod...