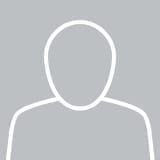
Carlos SantiagoTechnical University of Lisbon | UTL · Institute for Systems and Robotics (ISR)
Carlos Santiago
MSc Biomedical Engineering, PhD in Electric and Computer Engineering
About
39
Publications
6,513
Reads
How we measure 'reads'
A 'read' is counted each time someone views a publication summary (such as the title, abstract, and list of authors), clicks on a figure, or views or downloads the full-text. Learn more
483
Citations
Introduction
Publications
Publications (39)
In the field of 3D Human Pose Estimation (HPE), accurately estimating human pose, especially in scenarios with occlusions, is a significant challenge. This work identifies and addresses a gap in the current state of the art in 3D HPE concerning the scarcity of data and strategies for handling occlusions. We introduce our novel BlendMimic3D dataset,...
Providing visual cues to justify the decisions of deep neural networks contributes significantly to increase their explainability. Typical strategies to provide explanations rely on saliency or attention maps that may not be easy to interpret. Moreover, the actual decision-making process is still a black-box. This paper proposes to overcome these l...
Intelligent agents are showing increasing promise for clinical decision-making in a variety of healthcare settings. While a substantial body of work has contributed to the best strategies to convey these agents’ decisions to clinicians, few have considered the impact of personalizing and customizing these communications on the clinicians’ performan...
The detection and classification of breast cancer lesions with computer-aided diagnosis systems has seen a huge boost in recent years due to deep learning. However, most works focus on 2D image modalities. Dealing with 3D MRI adds new challenges, such as data insufficiency and lack of local annotations. To handle these issues, this work proposes a...
Magnetic Resonance Imaging (MRI) is the recommended imaging modality in the diagnosis of breast cancer. However, each MRI scan comprises dozens of volumes for the radiologist to inspect, each providing its own set of information on the tissues being scanned. This paper proposes a multimodal framework that processes all the available MRI data in ord...
Exploiting well-labeled training sets has led deep learning models to astonishing results for counting biological structures in microscopy images. However, dealing with weak multi-rater annotations, i.e., when multiple human raters disagree due to non-trivial patterns, remains a relatively unexplored problem. More reliable labels can be obtained by...
Video analysis often requires locating and tracking target objects. In some applications, the localization system has access to the full video, which allows fine-grain motion information to be estimated. This paper proposes capturing this information through motion fields and using it to improve the localization results. The learned motion fields a...
In this paper, we developed BreastScreening-AI within two scenarios for the classification of multimodal beast images: (1) Clinician-Only; and (2) Clinician-AI. The novelty relies on the introduction of a deep learning method into a real clinical workflow for medical imaging diagnosis. We attempt to address three high-level goals in the two above s...
Explainability is a key feature for computer-aided diagnosis systems. This property not only helps doctors understand their decisions, but also allows less experienced practitioners to improve their knowledge. Skin cancer diagnosis is a field where explainability is of critical importance, as lesions of different classes often exhibit confounding c...
Deep neural networks have been tremendously successful at segmenting objects in images. However, it has been shown they still have limitations on challenging problems such as the segmentation of medical images. The main reason behind this lower success resides in the reduced size of the object in the image. In this paper we overcome this limitation...
Convolutional Neural Networks have produced state-of-the-art results for a multitude of computer vision tasks under supervised learning. However, the crux of these methods is the need for a massive amount of labeled data to guarantee that they generalize well to diverse testing scenarios. In many real-world applications, there is indeed a large dom...
In this research, we take an HCI perspective on the opportunities provided by AI techniques in medical imaging, focusing on workflow efficiency and quality, preventing errors and variability of diagnosis in Breast Cancer. Starting from a holistic understanding of the clinical context, we developed BreastScreening to support Multimodality and integr...
Monitoring vehicle flows in cities is crucial to improve the urban environment and quality of life of citizens. Images are the best sensing modality to perceive and assess the flow of vehicles in large areas. Current technologies for vehicle counting in images hinge on large quantities of annotated data, preventing their scalability to city-scale a...
The performance of deep learning (DL) models is highly dependent on the quality and size of the training data, whose annotations are often expensive and hard to obtain. This work proposes a new strategy to train DL models by Learning Optimal samples Weights (LOW), making better use of the available data. LOW determines how much each sample in a bat...
Monitoring vehicle flow in cities is a crucial issue to improve the urban environment and quality of life of citizens. Images are the best sensing modality to perceive and asses the flow of vehicles in large areas. Current technologies for vehicle counting in images hinge on large quantities of annotated data, preventing their scalability to city-s...
Object recognition and localization is still a very challenging problem, despite recent advances in deep learning (DL) approaches, especially for objects with varying shapes and appearances. Statistical models, such as an Active Shape Model (ASM), rely on a parametric model of the object, allowing an easy incorporation of prior knowledge about shap...
Achieving robust object segmentations requires the ability
to discard outliers (invalid observations) in the segmentation
process. When considering an object model, such as an active
shape model (ASM), described by a set of points, a one-to-one
mapping from one model point to one valid observation
would be ideal. However, in general, a one-to-many...
Object segmentation is still an active topic that is highly visited in image processing and computer vision communities. This task is challenging due not only to difficult image conditions (e.g., poor resolution or contrast), but also to objects whose appearance vary significantly. This paper visits the Active Shape Model (ASM) that has become a wi...
Background and objective:
The segmentation of the left ventricle (LV) in cardiac magnetic resonance imaging is a necessary step for the analysis and diagnosis of cardiac function. In most clinical setups, this step is still manually performed by cardiologists, which is time-consuming and laborious. This paper proposes a fast system for the segment...
Three-dimensional active shape models use a set of annotated volumes to learn a shape model. Using unique landmarks to define the surface models in the training set, the shape model is able to learn the expected shape and variation modes of the segmentation. This information is then used during the segmentation process to impose shape constraints....
3D active shape models perform the estimation of deformable surfaces in 3D volumes using landmark statistics. First, the average shape and deformation modes are obtained from a set of annotated landmarks (training set). Then, these statistics are used to segment new volumes, involving the estimation of the alignment parameters and deformation coeff...
The 3D segmentation of endocardium of the left ventricle (LV) in cardiac MRI volumes is a challenging problem due to the intrinsic properties of this image modality. Typically, the object shape and position are estimated to fit the observed features collected from the images. The difficulty inherent to the LV segmentation in MRI is that the images...
3D Active shape models use a set of annotated volumes to learn a shape model. The shape model is defined by a fixed number of landmarks at specific locations and takes shape constraints into account in the segmentation process. A relevant problem in which these models can be used is the segmentation of the left ventricle in 3D MRI volumes. In this...
Statistical shape models have been extensively used in a wide range of applications due to their effectiveness in providing prior shape information for object segmentation problems. The most popular method is the Active Shape Model (ASM). However, accurately fitting the shape model to an object boundary under a cluttered environment is a challengin...
Active shape models (ASM) have been extensively used in object segmentation problems because they constrain the solution, using shape statistics. However, accurately fitting an ASM to an image prone to outliers is difficult and poor results are often obtained. To overcome this difficulty we propose a robust algorithm based on the Expectation-Maximi...
The segmentation of the left ventricle (LV) is an important task to assess the cardiac function in ultrasound images of the heart. This paper presents a novel methodology for the segmentation of the LV in three-dimensional (3-D) echocardiographic images based on the probabilistic data association filter (PDAF). The proposed methodology begins by in...
Statistical shape models have been extensively used in several image analysis problems, providing accurate estimates of object boundaries. However, their performance degrades if the object of interest is surrounded by a cluttered background, and the features extracted from the image contain outliers. Under these assumptions, most deformable models...
The segmentation of the left ventricle (LV) is an important tool to assess the cardiac function in ultrasound images of the heart. This paper presents a methodology for the segmentation of the LV in 3D echocardiography that is based on the probabilistic data association filter (PDAF). The proposed methodology comprises the following feature hierarc...
Finding correspondences between contour points in consecutive frames is crucial for the left ventricular motion analysis. In many medical applications, point correspondences can be determined by using distinctive anatomical features, called anatomical landmarks. However, in the case of cardiac images, these landmarks are scarce and insufficient for...
This paper presents a novel bottom-up deformable-based model for the segmentation of the Left Ventricle (LV) in 3D ultrasound data. The methodology presented here is based on Probabilistic Data Association Filter (PDAF). The main steps that characterize the proposed approach can be summarized as follows. After a rough initialization given by the us...
Deformable models are useful tools to extract shape information from images and video sequences. However, the model has to be initialized in the vicinity of the object boundary, in order to foster convergence towards the desired features. This chapter describes four methods which alleviate this restriction. Despite their differences, they share thr...
The segmentation of the left ventricle (LV) in echocardiographic data has proven itself a useful methodology to assess cardiac function and to detect abnormalities. Traditionally, cardiologists segment the LV border at the end-systolic and end-diastolic phases to determine the ejection fraction. However, the manual segmentation of the LV is a tedio...